AI will make investing in stocks harder as GPT-4 makes better forecasts than human analysts
Researchers have found that large language models can outperform humans in forecasting company future earnings, but your job should be safe, for now.
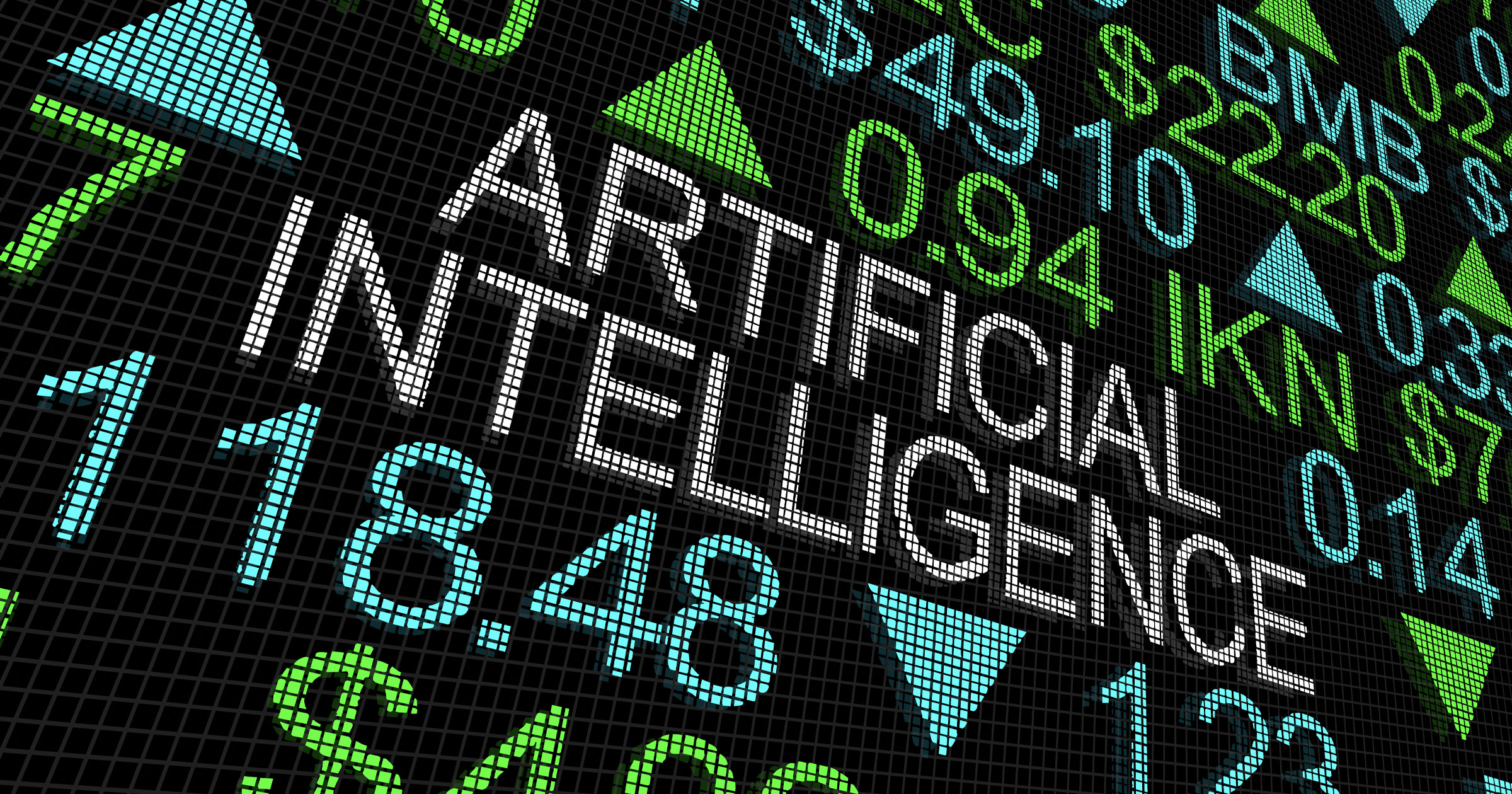
Disclaimer: Unless otherwise stated, any opinions expressed below belong solely to the author. The findings about GPT-4 performance vs. human analysts come from a recent paper from the University of Chicago, Booth School of Business
In a march for human jobs (as we are told, at least) Large Language Models have seemingly made another major stride, with OpenAI’s GPT-4 (the model behind ChatGPT) outperforming humans in analysing financial statements and forecasting future earnings of companies.
“Even without any narrative or industry-specific information, the LLM outperforms financial analysts in its ability to predict earnings changes.”
The findings come from a working paper published by researchers at the University of Chicago, Booth School of Business, who evaluated the LLM’s performance in evaluating companies solely on the basis of numbers, without their names or any contextual information about them.
What’s more, GPT-4 was found to be as accurate as purpose-trained machine learning models used in financial analysis so far.
This sounds like bad news for all people working as analysts — and a huge win for regular folks who will now be able to use a simple, accessible AI chatbot to help them pick stocks.
Or is it?
Your job is safe – for now
Let’s start by comforting people in finance—your job is likely safe, at least for now. GPT- 4’s advantage over a median analyst was just a few percentage points.
While humans got their predictions about future earnings right between 53 to 57 per cent of the time, the AI model reached 60.
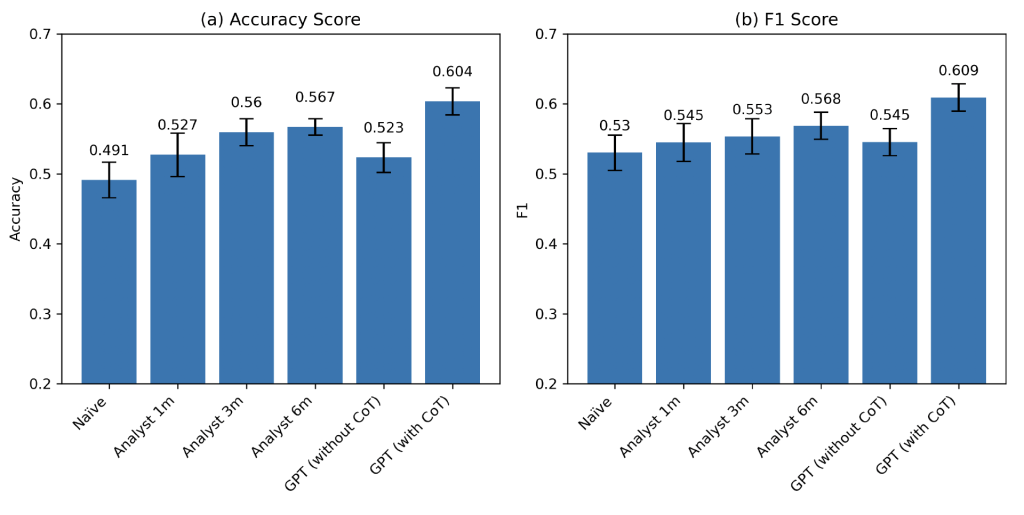
Of course, investing is a numbers game, and if you can improve your odds by a few points, it would be a no-brainer to take it. This is why the authors of the paper predict that:
“Taken together, our results suggest that LLMs may take a central role in decision-making.”
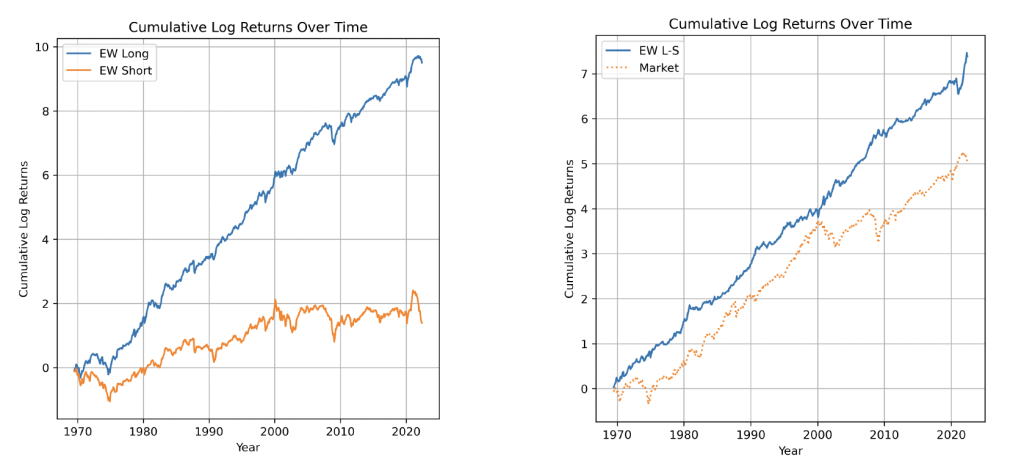
However, while the approach was tested on past data, going back to 1968, proving that decisions based on GPT-4s findings would generate above-average returns, it is impossible to know what it would look like if everybody was using the tool.
This is because the ubiquity of the solution could pretty much erase the advantages between different investors (both institutional and individual), effectively levelling the field, as everybody would be guided by the same, or highly similar, AI-generated recommendations.
This is why it might not be the silver bullet it seems to have been at the start, and why it can make investing much harder, not easier.
The shrinking alpha
In investing alpha is known as the rate of return above the market. In essence, it’s the measure of success of an investment against the average. Beating the market.
The alternative is simply to invest in the market. In stocks, this means parking your money with an index fund that tracks the overall performance of all companies and forgetting about it.
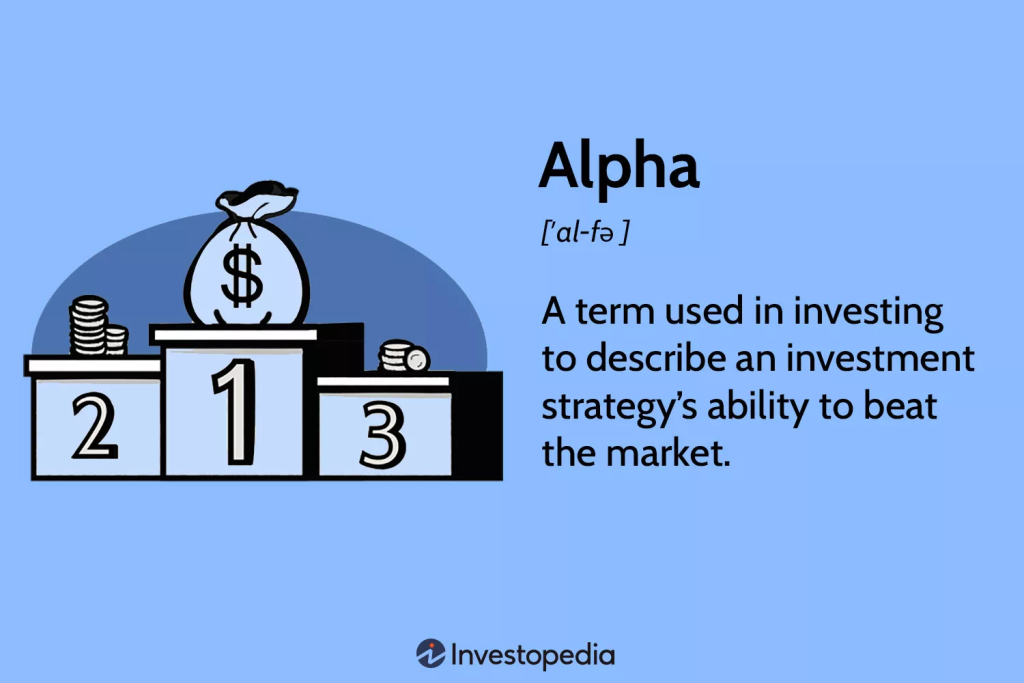
The whole point of financial analysis and portfolio management is to find the alpha, the value that is dissolved somewhere in the market, providing greater than expected returns year after year.
The fact of the matter is that the less information and more uncertainty there is, the better your chances of finding value that everybody else missed.
Conversely, the more we know and the better equipped we are, the fewer opportunities there are to discover something uniquely valuable.
This is why, while it may seem like a godsent to millions to have a tool to do the tiresome analysis of financial statements and come up with forecasts that guide your choice of stocks to invest in, the fact that so many people will instantly use this tool erases any advantage.
With more money flowing to certain companies on this basis, their prices will increase more quickly and are likely to be distributed among far too many investors to provide a meaningful return.
Moreover, there’s a genuine risk that such “AI signals” could contribute to the creation of bubbles, with far too many people following automated recommendations blindly, ending in greater losses following the inevitable burst.
The emergence of AI bots as financial advisors could make us outsource thinking to them, making us less risk-averse than it would be reasonable for us to be. After all, we can later blame it on the bot.
AI is making humans more valuable
The paradoxical outcome of these findings is that AI would make humans not less but more valuable – at least those who can make a difference.
After all, even the paper compared GPT-4s performance against the median, not the top-performing analysts (who aren’t revealed).
In other words, while AI is more accurate than humans, this doesn’t mean there are no humans who are better than AI.
Secondly, since it is only natural for us to use every tool available, the advantage of using them will quickly be levelled.
This means that making a difference that provides a better return will be down to humans, after all, even as the field for it becomes narrower. It may occur by improving the technology in private, in their own, purpose-built models (if possible), or by finding value in areas not easily analysed by machines (e.g. by judging the capacity of the team running a particular company, the strength of their experience, knowledge or intellectual property).
By taking up burdensome, repetitive tasks, intelligent, thinking machines are freeing us to do what we are born to excel at. And while it could mean fewer people will find employment in the disrupted industries, they will enjoy higher pay and better life satisfaction at work as a result.
As for the rest of us, we might be better off relying on them for our future investments.