A new use for AI: summarizing scientific research for seven-year-olds
tl;dr papers shows AI’s potential to condense research Continue reading…
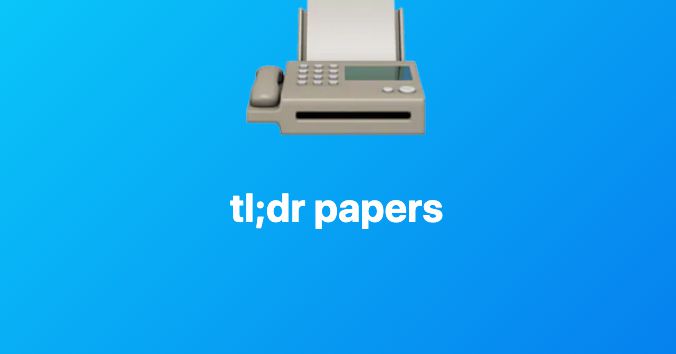
Academic writing often has a reputation for being hard to follow, but what if you could use machine learning to summarize arguments in scientific papers so that even a seven-year-old could understand them? That’s the idea behind tl;dr papers — a project that leverages recent advances in AI language processing to simplify science.
Work on the site began two years ago by university friends Yash Dani and Cindy Wu as a way to “learn more about software development,” Dani tells The Verge, but the service went viral on Twitter over the weekend when academics started sharing AI summaries of their research. The AI-generated results are sometimes inaccurate or simplified to the point of idiocy. But just as often, they are satisfyingly and surprisingly concise, cutting through academic jargon to deliver what could be mistaken for child-like wisdom.
Take this summary of a paper by Professor Michelle Ryan, director of the Global Institute for Women’s Leadership at the Australian National University. Ryan has written on the concept of the “glass cliff,” a form of gender discrimination in which women are placed in leadership roles at times when institutions are at their greatest risk of failure. The AI summary of her work? “The glass cliff is a place where a lot of women get put. It’s a bad place to be.”
“It is just excellent,” as Ryan put it.
Ryan tells The Verge the summary was “accurate and pithy,” though it did elide a lot of nuances around the concept. In part, this is because of a crucial caveat: tl;dr papers only analyzes the abstract of a scientific paper, which is itself a condensed version of a researcher’s argument. (Being able to condense an entire paper would be a much greater challenge, though it’s something machine learning researchers are already working on.)
Ryan says that although tl;dr papers is undoubtedly a very fun tool, it also offers “a good illustration of what good science communication should look like.” “I think many of us could write in a way that is more reader-friendly,” she says. “And the target audience of a second-grader is a good place to start.”
Zane Griffin Talley Cooper, a PhD candidate at the Annenberg School for Communication at the University of Pennsylvania, described the AI summaries as “refreshingly transparent.” He used the site to condense a paper he’d written on “data peripheries,” which traces the physical history of materials essential to big data infrastructure. Or, as tl;dr papers put it:
“Big data is stored on hard disk drives. These hard disk drives are made of very small magnets. The magnets are mined out of the ground.“
Cooper says although the tool is a “joke on the surface,” systems like this could have serious applications in teaching and study. AI summarizers could be used by students as a way into complex papers, or they could be incorporated into online journals, automatically producing simplified abstracts for public consumption. “Of course,” says Cooper, this should be only done “if framed properly and with discussion of limitations and what it means (both practically and ethically) to use machine learning as a writing tool.”
These limitations are still being explored by the companies that make these AI systems, even as the software is incorporated into ever-more mainstream tools. tl;dr papers itself was run on GPT-3, which is one of the best-known AI writing tools and is made by OpenAI, a combined research lab and commercial startup that works closely with Microsoft.
Microsoft has used GPT-3 and its ilk to build tools like autocomplete software for coders and recently began offering businesses access to the system as part of its cloud suite. The company says GPT-3 can be used to analyze the sentiment of text, generate ideas for businesses, and — yes — condense documents like the transcripts of meetings or email exchanges. And already, tools similar to GPT-3 are being used in popular services like Google’s Gmail and Docs, which offer AI-powered autocomplete features to users.
But the deployment of these AI-language systems is controversial. Time and time again, it’s been shown that these tools encode and amplify harmful language based on their training data (which is usually just vast volumes of text scraped off the internet). They repeat racist and sexist stereotypes and slurs and may be biased in more subtle ways, too.
A different set of worries stems from the inaccuracy of these systems. These tools only manipulate language on a statistical level: they have no human-equivalent understanding of what they’re “reading,” and this can lead to some very basic mistakes. In one notorious example that surfaced last year, Google search — which uses AI to summarize search topics — provided misleading medical advice to a query asking what to do if someone suffers a seizure. While last December, Amazon’s Alexa responded to a child asking for a fun challenge to do by telling them to touch a penny to the exposed prongs of a plug socket.
The specific danger to life posed by these scenarios is unusual, but they offer vivid illustrations of the structural weaknesses of these models. Jathan Sadowski, a senior research fellow in the Emerging Technologies Research Lab at Monash University, was another academic entertained by tl;dr papers’ summary of his research. He says AI systems like this should be handled with care, but they can serve a purpose in the right context.
“Maybe one day [this technology will] be so sophisticated that it can be this automated research assistant who is going and providing you a perfect, accurate, high quality annotated bibliography of academic literature while you sleep. But we are extremely far from that point right now,” Sadowski told The Verge. “The real, immediate usefulness from the tool is — first and foremost — as a novelty and joke. But more practically, I could see it as a creativity catalyst. Something that provides you this alien perspective on your work.”
Sadowski says the summaries provided by tl;dr papers often have a sort of “accidental wisdom” to them — a byproduct, perhaps, of machine learning’s inability to fully understand language. In other scenarios, artists have used these AI tools to write books and music, and Sadowski says a machine’s perspective could be useful for academics who’ve burrowed too deep in their subject. “It can give you artificial distance from a thing you’ve spent a lot of time really close to, that way you can maybe see it in a different light,” he says.
In this way, AI systems like tl;dr papers might even find a place similar to tools designed to promote creativity. Take, for example, “Oblique Strategies,” a deck of cards created by Brian Eno and Peter Schmidt. It offers pithy advice to struggling artists like “ask your body” or “try faking it!” Are these words of wisdom imbued with deep intelligence? Maybe, maybe not. But their primary role is to provoke the reader into new patterns of thinking. AI could offer similar services, and indeed, some companies already sell AI creative writing assistants.
Unfortunately, although tl;dr papers has had a rapturous reception among the academic world, its time in the spotlight looks limited. After going viral this weekend, the website has been labeled “under maintenance,” and the site’s creators say they have no plans to maintain it in the future. (They also mention that other tools have been built that perform the same task.)
Dani told The Verge that tl;dr papers “was designed to be an experiment to see if we can make learning about science a little easier, more fun, and engaging.” He says: “I appreciate all of the attention the app has received and thank all of the people who have tried it out [but] given this was always intended to be an educational project, I plan to sunset tl;dr papers in the coming days to focus on exploring new things.”