AI-Powered Threat Detection: Leveraging Platform Engineering to Develop AI Algorithms That Can Identify and Respond to Advanced Threats in Real Time
If your organization is the victim of a cybersecurity breach on January 1, 2025, it will take you until July 13, 2025 — or 194 days — to evaluate the extent of the compromise and develop a sustainable plan...
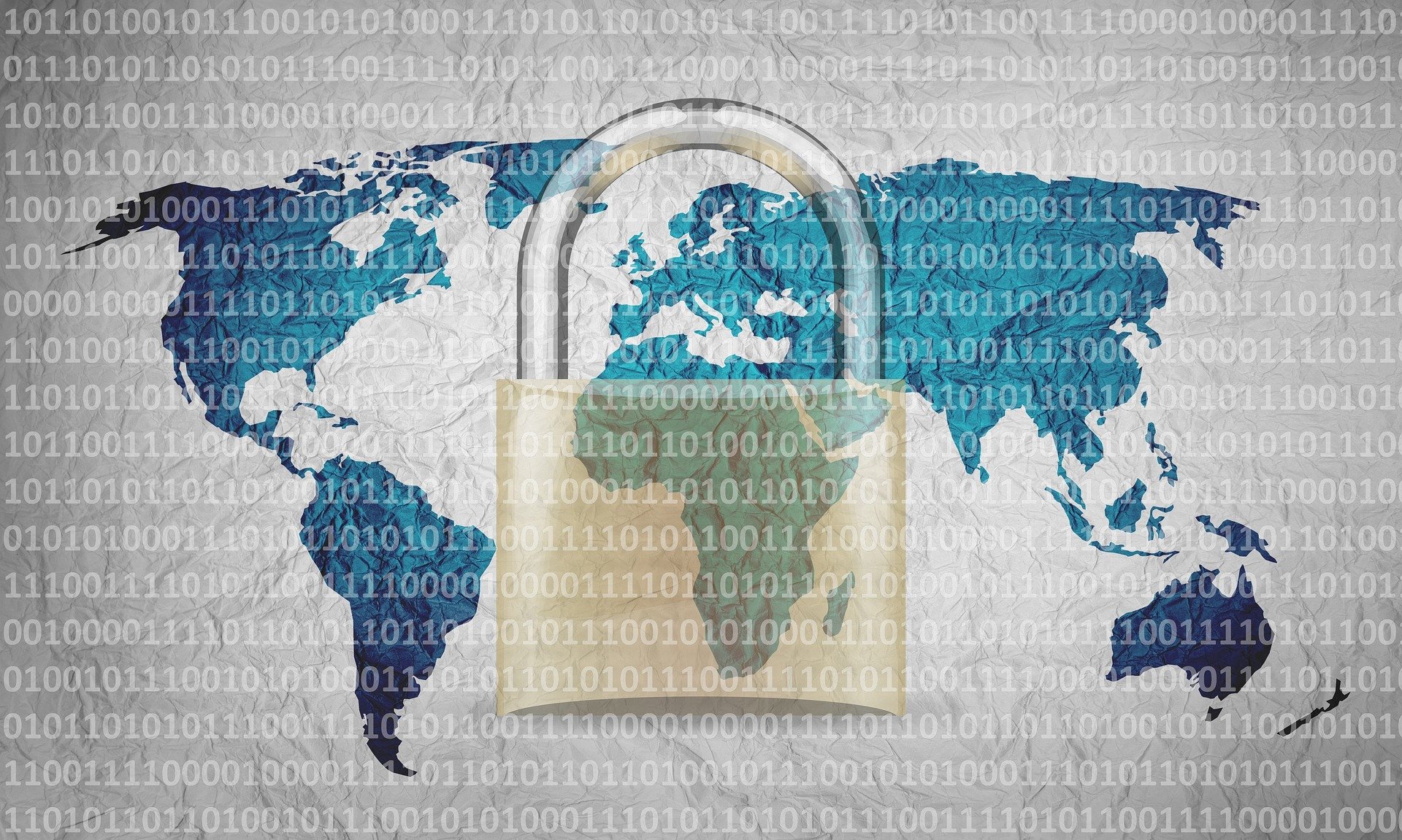
If your organization is the victim of a cybersecurity breach on January 1, 2025, it will take you until July 13, 2025 — or 194 days — to evaluate the extent of the compromise and develop a sustainable plan to address the breach. According to a recent IBM report, this is how long it took companies, on average, to identify a breach.
A delay of that length can lead to significant harm to any business, and the longer the activity goes undetected, the more time hackers have to harvest data and exploit further vulnerabilities. For the organization that has been breached, a delay can translate to regulatory fines, increased liability insurance premiums, and, more importantly, the loss of consumer trust.
While developers are trying to leverage artificial intelligence (AI) to reduce detection delays and proactively monitor the entire surface attack area in real time, they are encountering severe limitations in using third-party SaaS solutions or agents on their existing technology stack.
Using AI for anomaly detection
One key function AI algorithms contribute to cybersecurity is anomaly detection. An organization’s network activity typically follows consistent patterns, wherein employees access the network from specific entry points during work hours, and data is moved at certain rates between certain points. As organizations grow, activity scales in a predictable way.
Anomaly detection is an effective element of cybersecurity because attacks typically violate normal activity patterns. They may involve activity during off-hours, uncharacteristic data transfers, or connections with unfamiliar IP addresses.
However, detecting that type of activity is a challenge for manual systems. Even small organizations generate a significant amount of network activity, and identifying anomalies manually is like finding a needle in a haystack. AI, with its speed and accuracy in data analytics, can power systems that identify unusual patterns in network traffic in real time.
Using AI to enhance threat intelligence
Knowing what an attack will look like is an essential component of cybersecurity. Understanding new attack techniques is critical for developing and deploying effective controls. Responding to shifts in attack trends, such as the increase in phishing attacks that occurred during the COVID-19 pandemic, which inspired a shift to remote work, is also important.
Threat intelligence improves security by helping organizations train, detect, and maintain cybersecurity systems. The more up-to-date an organization’s intelligence, the better prepared it will be. However, keeping up with evolving threats can be challenging, even for well-resourced organizations.
AI can monitor threat intelligence feeds to identify trends and analyze how they could affect an organization. For example, AI can act as a threat detector by filtering threat intelligence for specific industries, regions, or businesses of certain sizes to help organizations better anticipate how to strengthen their controls. With advanced systems, threat analysis can automatically inform the AI algorithms used to detect anomalies, creating a heightened awareness of the type of activity that could result from new trends.
Using AI for behavioral analysis
An organization’s employees are the cybersecurity weakness most often exploited by hackers. Some reports say as many as 98 percent of cyber attacks use social engineering schemes to compromise controls. Consequently, monitoring and analyzing human behavior can improve security by revealing when hackers are targeting employees or have gained access to employee login credentials.
AI-driven behavioral analysis begins with establishing a baseline for employee activity. By examining login times, device usage patterns, and data access habits, AI platforms can create definitions of normal behavior. When activity deviates from that baseline, the platform can alert security personnel.
Behavioral analysis can identify sophisticated attacks, such as zero-day and advanced persistent threats, that often go unnoticed. Because it responds to deviations from regular activity rather than focusing on identified attack patterns, behavioral analysis can also safeguard organizations from unknown threats.
Using AI for automated responses
Using AI as a threat detector is only part of an effective cybersecurity response. Organizations must also respond quickly to cut off attackers. The enhanced speed that AI brings to attack detection is wasted if it doesn’t prompt a rapid response.
AI can help to facilitate a complete security framework by automating incident response procedures. Rather than simply generating a notification when suspicious activity is detected, AI can initiate processes that isolate infected systems or lock affected accounts.
Automated responses can also boost security when used in tandem with security audits. If AI detects that security patches are outdated, for example, it can automatically update them. Leveraging AI in this way allows organizations to be proactive about security rather than waiting for signs that hackers are exploiting vulnerabilities.
The US Federal Bureau of Investigation (FBI) recently warned organizations to expect cyber attacks to become more sophisticated as cybercriminals begin leveraging AI. “AI provides augmented and enhanced capabilities to schemes that attackers already use and increases cyber-attack speed, scale, and automation,” it said.
As organizations embrace the power of AI algorithms, they gain the advanced capabilities needed to repel the advanced attacks the FBI is seeing. AI transforms security into an intelligent response that rapidly identifies schemes and automatically addresses them.