How AI Is Changing The Way We Measure Success In Digital Advertising via @sejournal, @LisaRocksSEM
AI is transforming PPC metrics, shifting from clicks to predictive modeling, automated bidding, and advanced attribution for smarter, more effective ad campaigns. The post How AI Is Changing The Way We Measure Success In Digital Advertising appeared first on...
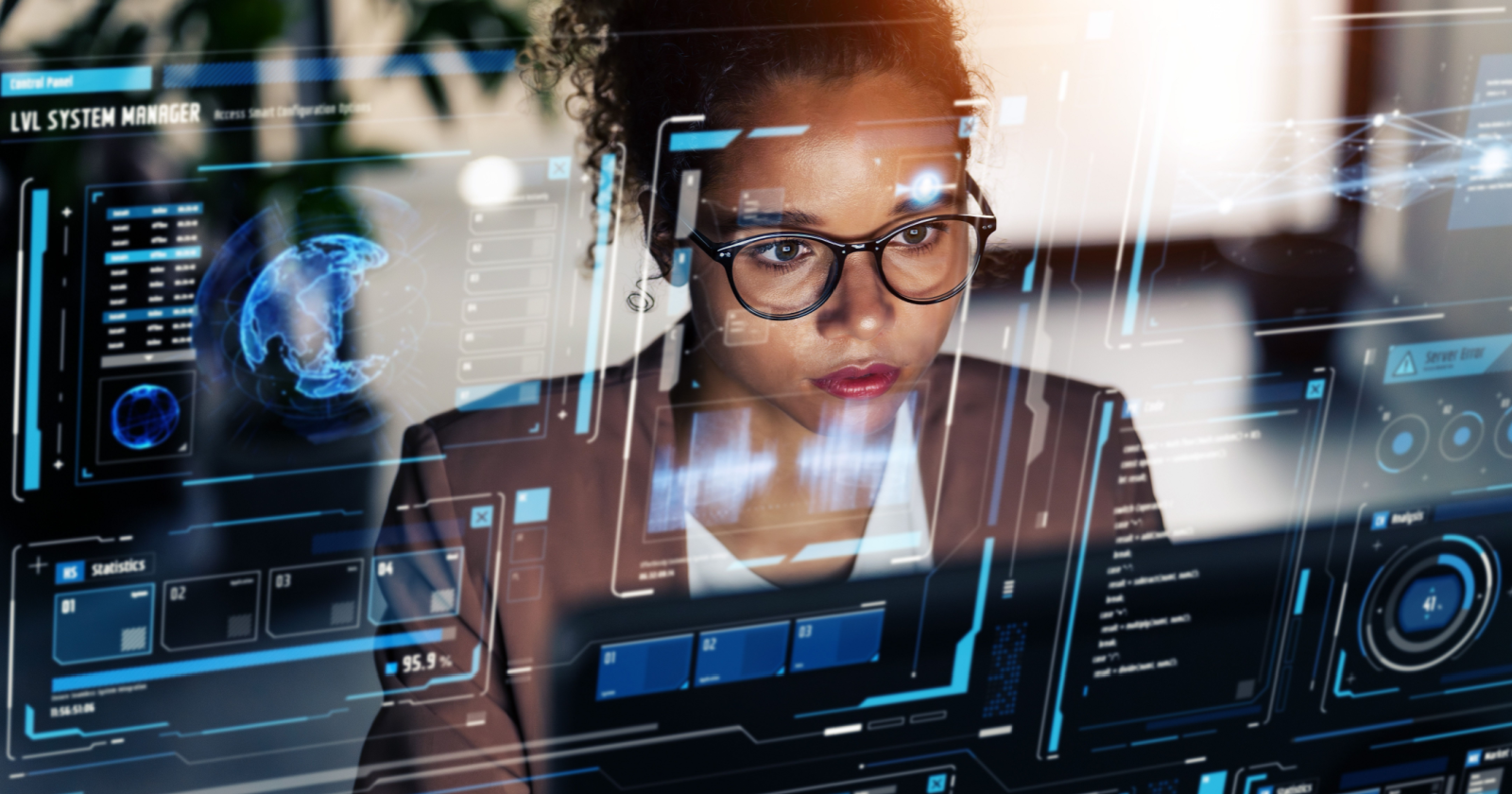
Success in PPC has historically been measured using performance indicators like click-through rates (CTR), cost per acquisition (CPA), and return on ad spend (ROAS).
However, with the rise of AI, new technologies are having an impact on how we approach and measure performance and success, causing a major change in customer behavior.
From Click-Based Metrics To Predictive Performance Modeling
PPC has relied heavily on click-based metrics, it’s even in the name “pay-per-click.” This has always provided immediate but narrow insights.
AI changes this by integrating predictive performance modeling: Machine learning algorithms analyze historical data to predict which campaigns will drive conversions.
Predictive modeling in AI-powered marketing is revolutionizing how advertisers allocate their precious resources by identifying high-converting audience segments before campaigns even launch.
Instead of reacting to past performance, AI-driven predictive analytics helps businesses forecast:
Future customer behaviors based on past interactions. The likelihood of conversion for different audience segments. The optimal bid adjustments for different times of day or geographies.This allows a more in-depth and detailed budget allocation and performance optimizations beyond simple impressions or clicks.
Quality Score 2.0 – AI-Driven Relevance Metrics
Google’s long-standing Quality Score is based on expected CTR, ad relevance, and landing page experience.
With the current tech advancements, it no longer provides a complete picture of user intent or engagement. AI provides a more advanced approach that some in the industry refer to as “Quality Score 2.0.”
AI-powered relevance metrics now analyze:
Deeper contextual signals beyond keywords, including sentiment analysis and user intent. Engagement and behavior patterns to determine the likelihood of conversions. Automated creative testing and adaptive learning to refine ad messaging in real-time.Google’s AI-driven Performance Max campaigns now use advanced machine learning techniques to optimize ad relevance, suggesting that the traditional Quality Score may soon be obsolete.
Automated Bidding & AI-Driven KPIs
Automated “smart” bidding has changed the way advertisers manage campaign performance.
Manual bid strategies have always required constant monitoring, now AI dynamically adjusts bids based on real-time data signals such as:
User device, location, and browsing behavior. Time-of-day performance variations. Probability of conversion based on previous engagement.Automated bidding strategies like Maximize Conversion Value and Target ROAS are outperforming manual CPC approaches, increasing account efficiencies.
AI-driven key performance indicators (KPIs) are helping advertisers shift to goal-based strategies tied directly to revenue.
Campaigns hitting the revenue goals can be easily scaled, which is a big step in maximizing PPC investments.
The Rise Of New AI-Generated PPC Metrics
Beyond improving existing measurement models, AI is introducing entirely new ways to assess digital ad performance.
These AI-driven PPC metrics offer more holistic insights into customer engagement and lifetime value.
AI Attribution Modeling
Attribution has always been a challenge in PPC.
Traditional models like last-click and linear attribution often miss the full picture by giving all the credit to a single touchpoint, making it hard to understand how different interactions actually contribute to conversions.
AI-powered attribution models solve this by using machine learning to distribute credit across multiple interactions, including clicks, video views, offline actions, and cross-device conversions.
This approach captures the complete customer journey rather than just focusing on the last click interaction.
AI attribution models typically include:
Data-Driven Attribution: Measures the true impact of each interaction, whether it’s a click, view, or engagement. Dynamic Adaptation: Continuously adjusts as new data comes in to keep the model accurate and up-to-date. Cross-Channel Integration: Combines online and offline data to reduce gaps and blind spots in tracking.AI Attribution Modeling is a measurement tool and provides a comprehensive view of how interactions contribute to long-term value.
It is also a strategic approach that connects both Engagement Value Score (EVS) and Customer Lifetime Value (CLV).
EVS measures the depth and quality of interactions rather than just clicks, while CLV focuses on the long-term worth of a customer.
By combining AI attribution with EVS and CLV, marketers gain a deeper understanding of the customer journey and can optimize campaigns for both meaningful engagement and sustainable growth rather than just short-term conversions.
Let’s dive into these two more specific metrics.
Engagement Value Score (EVS)
A growing alternative to CTR, the EVS measures how meaningful an interaction is rather than just if a click occurred.
Unlike CTR, which assumes all clicks are valuable, EVS pinpoints users who genuinely engage with your content.
To measure EVS, combine different engagement signals into one score. Start with your key engagement actions, like:
Time Spent on Site: How long users stay on your pages. Multi-Touch Interactions: Video views, chatbot conversations, or content consumption. Behavioral Indicators of Intent: Scroll depth or repeat visits.After assigning points to each action, create a custom metric in Google Analytics 4 that calculates the total EVS score from these individual actions and integrates into the Google Ads account.
Implementation Steps:
Create Events: Set up custom engagement events with conditions that match high EVS behaviors. Mark as Key Events: After creating these custom events, mark them as ket events in GA4. Import to Google Ads: Once the custom conversion is set up in GA4, import it into Google Ads. Align Bidding Strategies: Use automated bidding strategies that optimize for conversions rather than just clicks.By using this EVS methodology, Google Ads can optimize campaigns not just for clicks, but for meaningful interactions that drive high value.
Customer Lifetime Value (CLV)
Rather than optimizing for one-time conversions, Customer Lifetime Value (CLV) focuses on the long-term value of a customer.
AI-driven CLV measurement looks beyond quick wins and digs into the total worth of a customer over their entire relationship with your brand.
It’s similar to using EVS in that is focuses on meaningful interactions rather than quick clicks.
To measure CLV accurately, AI models analyze key data points like:
Past Purchase Behavior: Predicts future spend based on historical transactions. Churn Risk and Retention Probability: Identifies how likely a customer is to leave or stay. Cross-Channel Interactions: Tracks engagement across social media, email, and customer support.Just like EVS, CLV requires combining multiple signals into one clear metric. After gathering these data points, create a custom metric in GA4 that calculates the total CLV from individual interactions.
Implementation Steps:
Create Events: Set up custom engagement events for key behaviors (like repeat purchases or social interactions). Mark as Key Events: Once created, mark these events as key events in GA4. Import to Google Ads: Bring the custom conversion data into Google Ads to guide bidding strategies. Optimize with AI: Use automated bidding and predictive analytics to prioritize high-CLV customers.AI-powered CLV analysis is gaining traction as businesses move toward sustainable, long-term growth strategies rather than chasing short-term conversions.
Take a scientific deep dive into this topic, including risk-adjusted CLV, here.
Challenges And Considerations
While AI-driven measurement is transforming PPC advertising, it is not without its challenges. Decision-makers need to consider the following:
Data Privacy & Compliance
AI’s ability to collect and analyze large amounts of user data raises concerns about privacy and compliance.
General Data Protection Regulation (GDPR) and California Consumer Privacy Act (CCPA) are data privacy laws that regulate how businesses collect, store, and use personal information from consumers.
With these regulations, advertisers must balance data-driven insights with ethical and legal responsibilities. AI-powered models should prioritize anonymized data and ensure transparency in data usage.
AI Accuracy
Machine learning models rely on historical data, which can sometimes lead to inaccuracies.
If an AI model is trained on outdated or incomplete data, it can result in poor decision-making. Human oversight is needed to reduce these risks.
Algorithmic Bias
AI models can sometimes reflect biases present in the data they are trained on.
If left unchecked, this can lead to skewed campaign recommendations that favor certain demographics over others. Businesses must check that AI tools are built with fairness and inclusivity in mind.
Interpreting AI-Generated Insights
AI provides highly complex data outputs, which can be difficult for marketing teams to interpret.
Businesses should invest in AI literacy training for decision-makers and teams to ensure that insights are actionable and interpreted correctly.
Key Takeaways
AI is fundamentally changing how we measure success in PPC and digital advertising.
From predictive performance modeling to AI-driven attribution, CLV, and EVS, these advanced metrics are helping marketers move beyond basic clicks and short-term conversions.
Instead, they focus on deeper insights that drive sustainable growth and long-term value.
However, leveraging AI responsibly requires navigating challenges like data privacy, accuracy, algorithmic bias, and the complexity of interpreting insights.
Marketers must prioritize transparency, fairness, and continuous learning to make the most of these powerful tools.
The future of digital advertising lies in bringing together data insights and thoughtful strategy and sustaining that success over time.
More Resources:
Essential Guide To PPC Automation Layering: Boosting Efficiency And Effectiveness PPC Experts On AI In PPC: Potential & Limitations PPC Trends 2025Featured Image: metamorworks/Shutterstock