How to Do A/B Testing: 15 Steps for the Perfect Split Test
When marketers like us create landing pages, write email copy, or design call-to-action buttons, it can be tempting to use our intuition to predict what will make people click and connect.
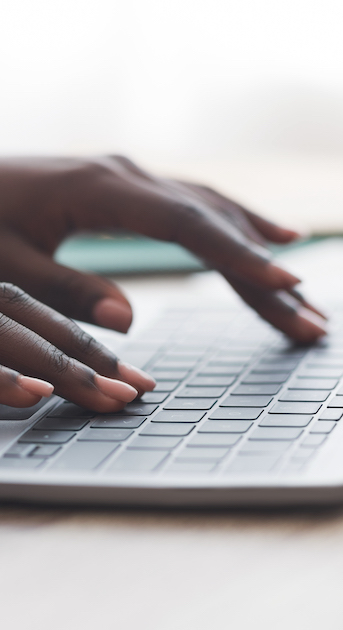
When marketers like us create landing pages, write email copy, or design call-to-action buttons, it can be tempting to use our intuition to predict what will make people click and connect. However, you’re much better off conducting A/B testing than basing marketing decisions off of a “feeling”, as this can be detrimental to your results. Keep reading to learn how to conduct the entire A/B testing process before, during, and after data collection so you can make the best decisions from your results. A/B testing, also known as split testing, is a marketing experiment wherein you split your audience to test a number of variations of a campaign and determine which performs better. In other words, you can show version A of a piece of marketing content to one half of your audience, and version B to another. A/B testing can be valuable because different audiences behave, well, differently. Something that works for one company may not necessarily work for another. In fact, conversion rate optimization (CRO) experts hate the term "best practices" because it may not actually be the best practice for you. But, this kind of testing can be complex if you’re not careful. Let’s go over how A/B testing works to ensure that you don’t make incorrect assumptions about what your audience likes. To run an A/B test, you need to create two different versions of one piece of content, with changes to a single variable. Then, you'll show these two versions to two similarly sized audiences and analyze which one performed better over a specific period of time (long enough to make accurate conclusions about your results). A/B testing helps marketers observe how one version of a piece of marketing content performs alongside another. Here are two types of A/B tests you might conduct in an effort to increase your website's conversion rate: Perhaps you want to see if moving a certain call-to-action (CTA) button to the top of your homepage instead of keeping it in the sidebar will improve its click-through rate. To A/B test this theory, you'd create another, alternative web page that uses the new CTA placement. The existing design with the sidebar CTA — or the "control" — is Version A. Version B with the CTA at the top is the "challenger." Then, you'd test these two versions by showing each of them to a predetermined percentage of site visitors. Ideally, the percentage of visitors seeing either version is the same. Learn how to easily A/B test a component of your website with HubSpot's Marketing Hub. Perhaps you want to find out if changing the color of your call-to-action (CTA) button can increase its click-through rate. To A/B test this theory, you'd design an alternative CTA button with a different button color that leads to the same landing page as the control. If you usually use a red call-to-action button in your marketing content, and the green variation receives more clicks after your A/B test, this could merit changing the default color of your call-to-action buttons to green from now on. To learn more about A/B testing, download our free introductory guide here. A/B testing has a multitude of benefits to a marketing team, depending on what it is you decide to test. Above all, though, these tests are valuable to a business because they're low in cost but high in reward. Let's say you employ a content creator with a salary of $50,000/year. This content creator publishes five articles per week for the company blog, totaling 260 articles per year. If the average post on the company's blog generates 10 leads, you could say it costs just over $192 to generate 10 leads for the business ($50,000 salary ÷ 260 articles = $192 per article). That's a solid chunk of change. Now, if you ask this content creator to spend two days developing an A/B test on one article, instead of writing two articles in that time period, you might burn $192 because you're publishing one fewer article. But if that A/B test finds you can increase each article's conversion rate from 10 to 20 leads, you just spent $192 to potentially double the number of customers your business gets from your blog. If the test fails, of course, you lost $192 — but now you can make your next A/B test even more educated. If that second test succeeds in doubling your blog's conversion rate, you ultimately spent $384 to potentially double your company's revenue. No matter how many times your A/B test fails, its eventual success will almost always outweigh the cost to conduct it. There are many types of split tests you can run to make the experiment worth it in the end. Here are some common goals marketers have for their business when A/B testing: Now, let's walk through the checklist for setting up, running, and measuring an A/B test. Follow along with our free A/B testing kit with everything you need to run A/B testing including a test tracking template, a how-to guide for instruction and inspiration, and a statistical significance calculator to see if your tests were wins, losses, or inconclusive. Let's cover the steps to take before you start your A/B test. As you optimize your web pages and emails, you might find there are a number of variables you want to test. But to evaluate how effective a change is, you'll want to isolate one "independent variable" and measure its performance. Otherwise, you can't be sure which variable was responsible for changes in performance. You can test more than one variable for a single web page or email — just be sure you're testing them one at a time. To determine your variable, look at the elements in your marketing resources and their possible alternatives for design, wording, and layout. Other things you might test include email subject lines, sender names, and different ways to personalize your emails. Keep in mind that even simple changes, like changing the image in your email or the words on your call-to-action button, can drive big improvements. In fact, these sorts of changes are usually easier to measure than the bigger ones. Note: There are some times when it makes more sense to test multiple variables rather than a single variable. This is a process called multivariate testing. If you're wondering whether you should run an A/B test versus a multivariate test, here's a helpful article from Optimizely that compares the two processes. Although you'll measure several metrics during any one test, choose a primary metric to focus on before you run the test. In fact, do it before you even set up the second variation. This is your "dependent variable," which changes based on how you manipulate the independent variable. Think about where you want this dependent variable to be at the end of the split test. You might even state an official hypothesis and examine your results based on this prediction. If you wait until afterward to think about which metrics are important to you, what your goals are, and how the changes you're proposing might affect user behavior, then you might not set up the test in the most effective way. You now have your independent variable, your dependent variable, and your desired outcome. Use this information to set up the unaltered version of whatever you're testing as your control scenario. If you're testing a web page, this is the unaltered page as it exists already. If you're testing a landing page, this would be the landing page design and copy you would normally use. From there, build a challenger — the altered website, landing page, or email that you’ll test against your control. For example, if you're wondering whether adding a testimonial to a landing page would make a difference in conversions, set up your control page with no testimonials. Then, create your challenger with a testimonial. For tests where you have more control over the audience — like with emails — you need to test with two or more audiences that are equal in order to have conclusive results. How you do this will vary depending on the A/B testing tool you use. If you're a HubSpot Enterprise customer conducting an A/B test on an email, for example, HubSpot will automatically split traffic to your variations so that each variation gets a random sampling of visitors. How you determine your sample size will also vary depending on your A/B testing tool, as well as the type of A/B test you're running. If you're A/B testing an email, you'll probably want to send an A/B test to a subset of your list that is large enough to achieve statistically significant results. Eventually, you'll pick a winner and send the winning variation on to the rest of the list. (See "The Science of Split Testing" ebook at the end of this article for more on calculating your sample size.) If you're a HubSpot Enterprise customer, you'll have some help determining the size of your sample group using a slider. It'll let you do a 50/50 A/B test of any sample size — although all other sample splits require a list of at least 1,000 recipients. If you're testing something that doesn't have a finite audience, like a web page, then how long you keep your test running will directly affect your sample size. You'll need to let your test run long enough to obtain a substantial number of views. Otherwise, it will be hard to tell whether there was a statistically significant difference between variations. Once you've picked your goal metric, think about how significant your results need to be to justify choosing one variation over another. Statistical significance is a super important part of the A/B testing process that's often misunderstood. If you need a refresher, I recommend reading this blog post on statistical significance from a marketing standpoint. The higher the percentage of your confidence level, the more sure you can be about your results. In most cases, you'll want a confidence level of 95% minimum — preferably even 98% — especially if it was a time-intensive experiment to set up. However, sometimes it makes sense to use a lower confidence rate if you don't need the test to be as stringent. Matt Rheault, a senior software engineer at HubSpot, likes to think of statistical significance like placing a bet. What odds are you comfortable placing a bet on? Saying "I'm 80% sure this is the right design and I'm willing to bet everything on it" is similar to running an A/B test to 80% significance and then declaring a winner. Rheault also says you’ll likely want a higher confidence threshold when testing for something that only slightly improves conversion rate. Why? Because random variance is more likely to play a bigger role. "An example where we could feel safer lowering our confidence threshold is an experiment that will likely improve conversion rate by 10% or more, such as a redesigned hero section," he explained. "The takeaway here is that the more radical the change, the less scientific we need to be process-wise. The more specific the change (button color, microcopy, etc.), the more scientific we should be because the change is less likely to have a large and noticeable impact on conversion rate." Testing more than one thing for a single campaign — even if it's not on the same exact asset — can complicate results. For example, if you A/B test an email campaign that directs to a landing page at the same time that you’re A/B testing that landing page, how can you know which change caused the increase in leads? Let's cover the steps to take during your A/B test. To do an A/B test on your website or in an email, you'll need to use an A/B testing tool. If you're a HubSpot Enterprise customer, the HubSpot software has features that let you A/B test emails (learn how here), calls-to-action (learn how here), and landing pages (learn how here). For non-HubSpot Enterprise customers, other options include Google Analytics, which lets you A/B test up to 10 full versions of a single web page and compare their performance using a random sample of users. Timing plays a significant role in your marketing campaign’s results, whether it's time of day, day of the week, or month of the year. If you were to run Version A during one month and Version B a month later, how would you know whether the performance change was caused by the different design or the different month? When you run A/B tests, you'll need to run the two variations at the same time, otherwise you may be left second-guessing your results. The only exception here is if you're testing timing itself, like finding the optimal times for sending out emails. This is a great thing to test because depending on what your business offers and who your subscribers are, the optimal time for subscriber engagement can vary significantly by industry and target market. Again, you'll want to make sure that you let your test run long enough to obtain a substantial sample size. Otherwise, it'll be hard to tell whether there was a statistically significant difference between the two variations. How long is long enough? Depending on your company and how you execute the A/B test, getting statistically significant results could happen in hours ... or days ... or weeks. A big part of how long it takes to get statistically significant results is how much traffic you get — so if your business doesn't get a lot of traffic to your website, it'll take much longer for you to run an A/B test. Read this blog post to learn more about sample size and timing. A/B testing has a lot to do with quantitative data ... but that won't necessarily help you understand why people take certain actions over others. While you're running your A/B test, why not collect qualitative feedback from real users? One of the best ways to ask people for their opinions is through a survey or poll. You might add an exit survey on your site that asks visitors why they didn't click on a certain CTA, or one on your thank-you pages that asks visitors why they clicked a button or filled out a form. You might find, for example, that a lot of people clicked on a call-to-action leading them to an ebook, but once they saw the price, they didn't convert. That kind of information will give you a lot of insight into why your users are behaving in certain ways. Finally, let's cover the steps to take after your A/B test. Again, although you'll be measuring multiple metrics, keep your focus on that primary goal metric when you do your analysis. For example, if you tested two variations of an email and chose leads as your primary metric, don’t get caught up on open rate or click-through rate. You might see a high click-through rate and poor conversion rates, in which case you might end up choosing the variation that had a lower click-through rate in the end. Now that you've determined which variation performs the best, it's time to determine whether your results are statistically significant. In other words, are they enough to justify a change? To find out, you'll need to conduct a test of statistical significance. You could do that manually ... or you could just plug in the results from your experiment to our free A/B testing calculator. For each variation you tested, you'll be prompted to input the total number of tries, like emails sent or impressions seen. Then, enter the number of goals it completed — generally you'll look at clicks, but this could also be other types of conversions. The calculator will spit out the confidence level your data produces for the winning variation. Then, measure that number against the value you chose to determine statistical significance. If one variation is statistically better than the other, you have a winner. Complete your test by disabling the losing variation in your A/B testing tool. If neither variation is statistically better, you've just learned that the variable you tested didn't impact results, and you'll have to mark the test as inconclusive. In this case, stick with the original variation, or run another test. You can use the failed data to help you figure out a new iteration on your new test. While A/B tests help you impact results on a case-by-case basis, you can also apply the lessons you learn from each test and apply it to future efforts. For example, if you've conducted A/B tests in your email marketing and have repeatedly found that using numbers in email subject lines generates better clickthrough rates, you might want to consider using that tactic in more of your emails. The A/B test you just finished may have helped you discover a new way to make your marketing content more effective — but don't stop there. There’s always room for more optimization. You can even try conducting an A/B test on another feature of the same web page or email you just did a test on. For example, if you just tested a headline on a landing page, why not do a new test on body copy? Or a color scheme? Or images? Always keep an eye out for opportunities to increase conversion rates and leads. You can use HubSpot’s A/B Test Tracking Kit to plan and organize your experiments. As a marketer, you know the value of automation. Given this, you likely use software that handles the A/B test calculations for you — a huge help. But, after the calculations are done, you need to know how to read your results. Let’s go over how. The first step in reading your A/B test results is looking at your goal metric, which is usually conversion rate. After you’ve plugged your results into your A/B testing calculator, you’ll get two results for each version you’re testing. You’ll also get a significant result for each of your variations. By looking at your results, you’ll likely be able to tell if one of your variations performed better than the other. However, the true test of success is whether the results you have are statistically significant. This means that one variation performed better than the other at a significant level because, say, the CTA text was more compelling. Say, for example, Variation A had a 16.04% conversion rate and variation B had a 16.02% conversion rate, and your confidence interval of statistical significance is 95%. Variation A has a higher conversion rate, but the results are not statistically significant, meaning that Variation A won’t significantly improve your overall conversion rate. Regardless of significance, it's valuable to break down your results by audience segment to understand how each key area responded to your variations. Common variables for segmenting audiences are: Let’s go over some examples of A/B experiments you could run for your business. We’ve discussed how A/B tests are used in marketing and how to conduct one — but how do they actually look in practice? As you might guess, we run many A/B tests to increase engagement and drive conversions across our platform. Here are five examples of A/B tests to inspire your own experiments. Site search bars help users quickly find what they’re after on a particular website. HubSpot found from previous analysis that visitors who interacted with its site search bar were more likely to convert on a blog post. So, we ran an A/B test in an attempt to increase engagement with the search bar. In this test, search bar functionality was the independent variable and views on the content offer thank you page was the dependent variable. We used one control condition and three challenger conditions in the experiment. In the control condition (variant A), the search bar remained unchanged. In variant B, the search bar was made larger and more visually prominent, and the placeholder text was set to “search by topic.” Variant C appeared identical to variant B, but only searched the HubSpot Blog rather than the entire website. In variant D, the search bar was made larger but the placeholder text was set to “search the blog.” This variant also searched only the HubSpot Blog We found variant D to be the most effective: It increased conversions by 3.4% over the control and increased the percentage of users who used the search bar by 6.5%. HubSpot uses several CTAs for content offers in our blog posts, including ones in the body of posts as well as at the bottom of the page. We test these CTAs extensively for optimize their performance. For our mobile users, we ran an A/B test to see which type of bottom-of-page CTA converted best. For our independent variable, we altered the design of the CTA bar. Specifically, we used one control and three challengers in our test. For our dependent variables, we used pageviews on the CTA thank you page and CTA clicks. The control condition included our normal placement of CTAs at the bottom of posts. In variant B, the CTA had no close or minimize option. In variant D, we included an option to minimize the CTA with an up/down caret. Our tests found all variants to be successful. Variant D was the most successful, with a 14.6% increase in conversions over the control. This was followed by variant C with an 11.4% increase and variant B with a 7.9% increase. In another CTA experiment, HubSpot tested whether adding the word “free” and other descriptive language to author CTAs at the top of blog posts would increase content leads. Past research suggested that using “free” in CTA text would drive more conversions and that text specifying the type of content offered would be helpful for SEO and accessibility. In the test, the independent variable was CTA text and the main dependent variable was conversion rate on the content offer form. In the control condition, author CTA text was unchanged (see the orange button in the image below). In variant B, the word “free” was added to the CTA text. In variant C, descriptive wording was added to the CTA text in addition to “free.” Interestingly, variant B saw a loss in form submissions, down by 14% compared to the control. This was unexpected, since including "free" in content offer text is widely considered a best practice. Meanwhile, form submissions in variant C outperformed the control by 4%. It was concluded that adding descriptive text to the author CTA helped users understand the offer and thus made them more likely to download. To help users better navigate the blog, HubSpot tested a new Table of Contents (TOC) module. The goal was to improve user experience by presenting readers with their desired content more quickly. We also tested whether adding a CTA to this TOC module would increase conversions. The independent variable of this A/B test was the inclusion and type of TOC module in blog posts, and the dependent variables were conversion rate on content offer form submissions and clicks on the CTA inside the TOC module. The control condition did not include the new TOC module —control posts either had no table of contents, or a simple bulleted list of anchor links within the body of the post near the top of the article (pictured below). In variant B, the new TOC module was added to blog posts. This module was sticky, meaning it remained onscreen as users scrolled down the page. Variant B also included a content offer CTA at the bottom of the module. Variant C included an identical module to variant B but with the CTA removed. Both variants B and C did not increase the conversion rate on blog posts. The control condition outperformed variant B by 7% and performed equally with variant C. Also, few users interacted with the new TOC module or the CTA inside the module. To determine the best way of gathering customer reviews, we ran a split test of email notifications versus in-app notifications. Here, the independent variable was the type of notification and the dependent variable was the percentage of those who left a review out of all those who opened the notification. In the control, HubSpot sent a plain text email notification asking users to leave a review. In variant B, HubSpot sent an email with a certificate image including the user’s name. For variant C, HubSpot sent users an in app-notification. Ultimately, both emails performed similarly and outperformed the in-app notifications. About 25% of users who opened an email left a review versus the 10.3% who opened in-app notifications. Emails were also more often opened by users. A/B testing allows you to get to the truth of what content and marketing your audience wants to see. Learn how to best carry out some of the steps above using the free e-book below. Editor's note: This post was originally published in May 2016 and has been updated for comprehensiveness.What is A/B testing?
How does A/B testing Work?
Example 1: User Experience Test
Example 2: Design Test
A/B Testing in Marketing
How to Conduct A/B Testing
Before the A/B Test
1. Pick one variable to test.
2. Identify your goal.
3. Create a 'control' and a 'challenger.'
4. Split your sample groups equally and randomly.
5. Determine your sample size (if applicable).
6. Decide how significant your results need to be.
7. Make sure you're only running one test at a time on any campaign.
During the A/B Test
8. Use an A/B testing tool.
9. Test both variations simultaneously.
10. Give the A/B test enough time to produce useful data.
11. Ask for feedback from real users.
After the A/B Test
12. Focus on your goal metric.
13. Measure the significance of your results using our A/B testing calculator.
14. Take action based on your results.
15. Plan your next A/B test.
How to Read A/B Testing Results
1. Check your goal metric.
2. Compare your conversion rates.
3. Segment your audiences for further insights.
A/B Testing Examples
1. Site Search
2. Mobile CTAs
In variant C, mobile readers could close the CTA by tapping an X icon. Once it was closed out, it wouldn’t reappear.
3. Author CTAs
4. Blog Table of Contents
5. Review Notifications
Start A/B Testing Today
Originally published Mar 3, 2022 7:00:00 AM, updated March 03 2022