RAG AI: Breaking Down Data Silos to Reveal Unified Insights
If you were to conduct a survey asking workers what is the most important asset for businesses, they would most resoundingly say that data. However, some of the most structured businesses struggle with data silos, thus hindering their ability...
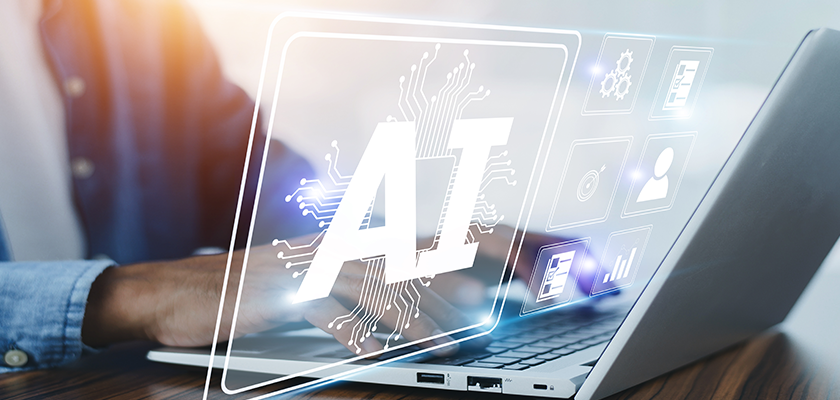
If you were to conduct a survey asking workers what is the most important asset for businesses, they would most resoundingly say that data.
However, some of the most structured businesses struggle with data silos, thus hindering their ability to derive meaningful insights that can benefit their growth and success. In fact, recent surveys from reputable outlets like Gartner, show that up to 80% of organizations report that data silos negatively impact their business operations.
That’s why it’s important to soak how silos can undermine businesses and how overcoming them is crucial for leveraging data to its fullest potential. If you’re curious to know more, this article will be of great use to you and we explore the significance of Retrieval-Augmented Generation AI and its involvement in transforming data integration so that companies can use it to their advantage.
The Evolution of Data Management: From On-Premises Databases to Cloud Computing
If we were to trace data management in its earliest days (or even current times for some companies), it can be characterized as a reliance on on-premises databases, where data is archived, maintained, and stored in physical servers within their own infrastructure. Though a relative means of security, this traditional method posed significant company challenges in terms of scalability, maintenance costs, and integration across different business units.
As the flourishing of technology has opened the floodgates to opportunities to store an unimaginable amount of data, cloud computing offers businesses the flexible and scalable storage solutions that businesses crave. Companies can now look to cloud-based platforms such as Amazon Web Services (AWS), Microsoft Azure, and Google Cloud Platform (GCP) to store and manage vast amounts of data without the constraints of physical infrastructure.
However, this goes without stating that this transition to cloud computing was met with its own set of challenges, primarily:
Data Fragmentation Across Multiple Cloud Services Security Concerns Integration ComplexityAccording to industry research, 94% of enterprises use cloud services, but 67% struggle with integrating data across multiple platforms, highlighting the ongoing complexity of cloud data management. This shift highlighted the need for more sophisticated integration techniques, setting the stage for data management solutions like Retrieval-Augmented Generation (RAG) AI.
The Role of APIs and Middleware in Legacy Data Integration
Before AI-driven solutions like RAG AI, businesses attempted to unify fragmented data using APIs (Application Programming Interfaces) and middleware. These technologies allowed different software applications to communicate and share data, bridging the gap between isolated systems.
While APIs and middleware provided partial solutions to crack the code on improving data silo issues, they had significant drawback, notably:
Manual Configuration & Maintenance: IT teams had to constantly update and manage APIs as systems evolved. Performance Bottlenecks: As data complexity increased, middleware solutions often struggled with latency issues in large-scale data processing. Lack of Contextual Awareness: Traditional integration methods lacked semantic understanding, making it difficult to extract meaningful insights from disparate data sources.How AI and Machine Learning Differ from Rule-Based Data Integration
AI and machine learning-based approaches dynamically analyze, retrieve, and process data, a stark contrast from traditional rule-based data integration which sets its foundation on predefined workflows and manual mappings.
Traditional Rule-Based Integration:
Requires manual configuration of rules and logic. Struggles with unstructured data (e.g., social media posts, emails). Inflexible and difficult to scale as data grows.AI-Driven Data Integration:
Uses Natural Language Processing (NLP) to extract meaning from unstructured data. Learns from past queries to improve accuracy over time. Can integrate real-time data retrieval with historical records.It’s only by comparatively looking at these two that we realize that RAG AI surpasses traditional approaches such as the automation of steps like discovery, retrieval, and contextualization of data makes the process smoother and enhances the ability to draw real-time insights.
Future Outlook and Emerging Capabilities
With its current deployment in many industries and sectors, including healthcare, logistics, and marketing, RAG AI will most likely be a mainstay. For companies to stay abreast with advancements and shifting priorities, it’s important that they cast a wide net on data that can be accessible and relevant without the pitfalls of security pinning them down.
RAG AI offers a powerful solution to the age-old problem of data silos. When used correctly, it can combine unified insights to drive more informed decision-making.
As AI-driven data management is roaring with opportunity, companies that use this solution to their advantage are only promising themselves a more righteous path to innovation and efficiency that will unmatch stubborn competitors who remain tepid to integrating newer data solutions.