SEO Reinvented: Responding To Algorithm Shifts via @sejournal, @pageonepower
Is your SEO strategy ready for the future? As Google’s algorithms evolve and consumer behavior shifts, it’s no longer enough to rely on isolated tactics. Today, effective SEO strategies must be part of a broader, integrated approach that includes...
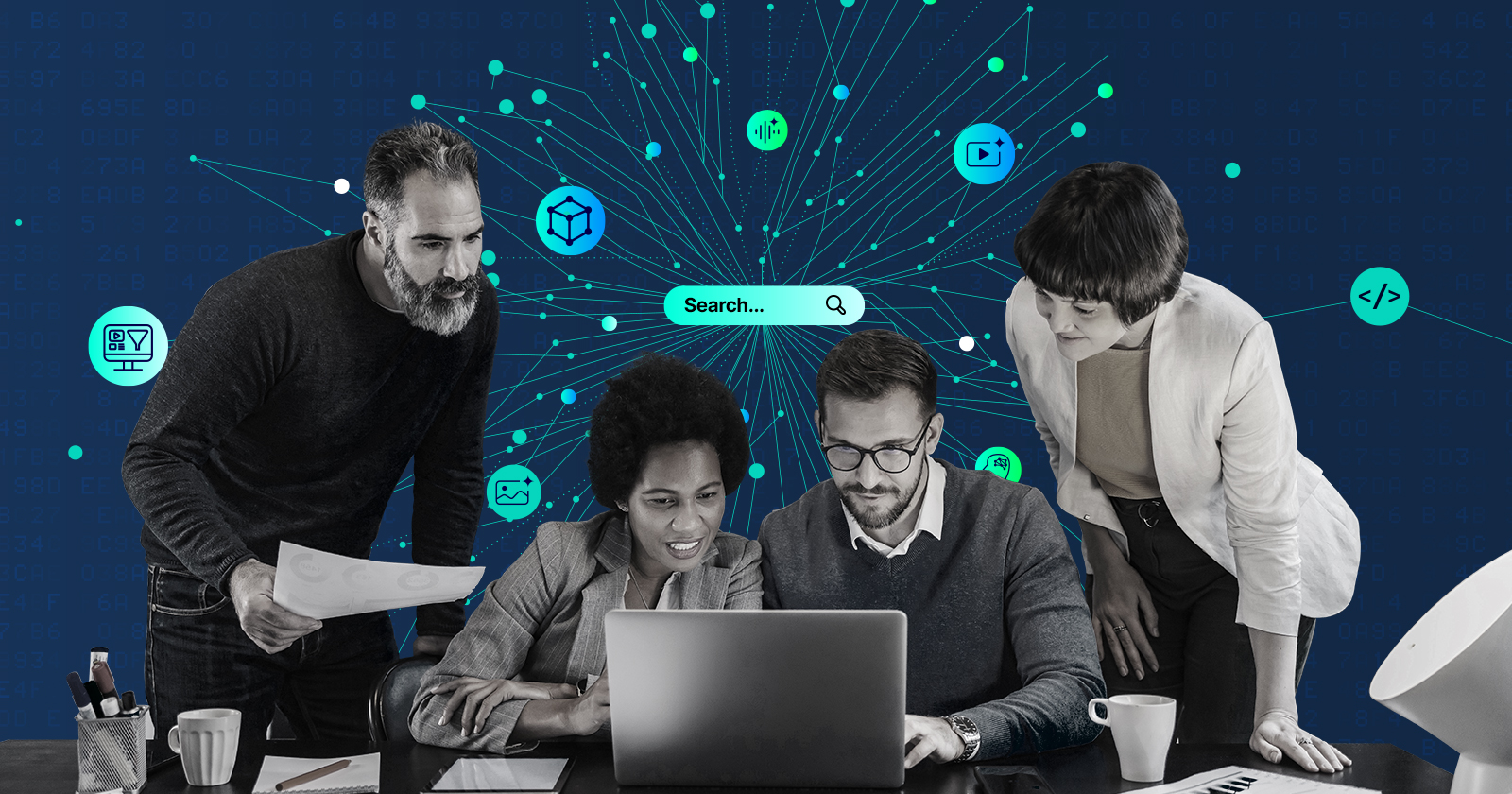
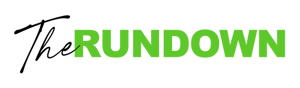
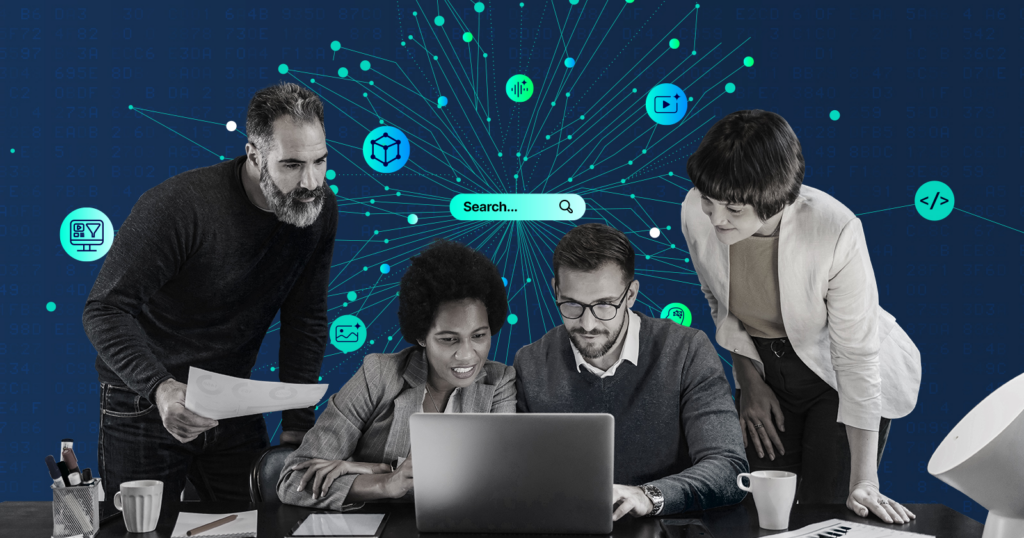
Is your SEO strategy ready for the future?
As Google’s algorithms evolve and consumer behavior shifts, it’s no longer enough to rely on isolated tactics. Today, effective SEO strategies must be part of a broader, integrated approach that includes content, public relations, and data-driven testing.
In this Rundown, we explore how search, SEO, content, and PR form the foundation of a comprehensive digital marketing stack. By understanding how these elements work together, you’ll be better equipped to adapt to changes in the search landscape and build sustainable success.
We’ll highlight key strategies that drive results across multiple channels and show you how to measure progress using the right metrics. Additionally, we’ll dive into the importance of testing theories before full implementation, helping you save on costs and boost your ROI.
Together, these tips, tactics, and insights are designed to ensure your brand can thrive in a constantly evolving digital ecosystem.

Katie Morton Editor in Chief, Search Engine Journal
Why Google's Latest Changes Call For A New Approach To SEO
Search and search behavior is changing. Learn how to adapt your SEO strategy to meet the challenges of today’s SERPs.
Page One Power
85 Reads
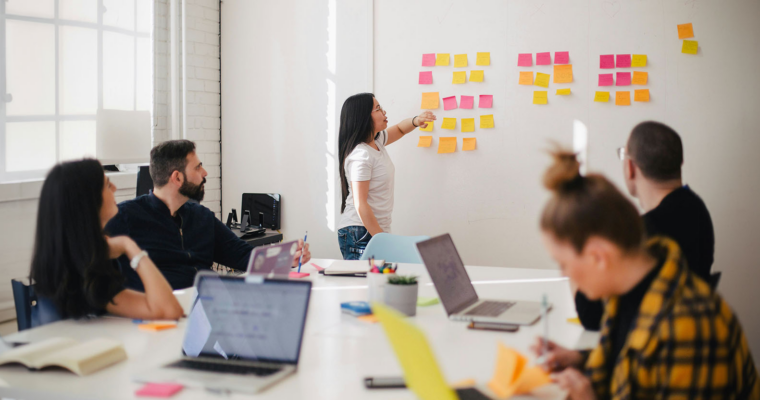
Recent developments at Google, including data leaks and the March 2024 core update, have changed how some practitioners think about SEO.
With engagement metrics taking center stage, SEO professionals must adapt their strategies to prioritize user interaction and satisfaction.
This article will examine how engagement metrics are reshaping key areas of SEO, with a focus on Conversion Rate Optimization (CRO), technical SEO, and strategic link building.
As we explore these topics, we’ll highlight how Page One Power’s expertise can help you navigate these changes.
Google’s Shift Towards Engagement Metrics
Recent leaks and updates suggest that Google is placing increased importance on user engagement signals.
Metrics such as click-through rates (CTR), time on site, bounce rates, and pages per session are likely more significant factors than previously thought.
The March 2024 core update further reinforced this trend, with sites providing exceptional user experiences seeing gains.
This shift calls for a more holistic approach to SEO.
Conversion Rate Optimization: The New Frontier Of SEO
With engagement metrics gaining prominence, CRO has become integral to SEO strategies. CRO directly impacts engagement signals that Google appears to be prioritizing.
How CRO Impacts Engagement Metrics
Improved Click-Through Rates: Optimizing titles, meta descriptions, and structured data can increase your CTR from search results, signaling to Google that your content is relevant and engaging. Reduced Bounce Rates: A well-optimized landing page that meets user expectations can lower bounce rates, indicating to Google that users find your content valuable. Increased Time on Site: Engaging, well-structured content and intuitive navigation can keep users on your site longer, another positive signal to Google. Higher Pages per Session: Internal linking and compelling content can encourage users to explore more of your site, increasing pages per session.CRO Techniques to Boost Engagement
A/B testing headlines, calls-to-action, and page layouts Improving site navigation and user flow Optimizing page load times for better user experience Personalizing content based on user behavior and preferences Implementing clear and compelling calls to actionFocusing on these CRO techniques can improve your conversion rates and send strong engagement signals to Google. Page One Power’s team of experts can help you develop and implement a CRO strategy that aligns with your goals.
Technical SEO: The Foundation of User Engagement
Technical SEO is vital to user engagement by ensuring that your website provides a seamless, fast, and accessible experience.
How Technical SEO Impacts Engagement Metrics
Site Speed: Faster-loading pages reduce bounce rates and increase time on site. Mobile Optimization: With mobile-first indexing, a mobile-friendly site is crucial for engagement across all devices. Site Architecture: A well-structured site improves crawlability and helps users find what they want, increasing pages per session. Schema Markup: Rich results can improve CTR from search results pages.Technical SEO Issues That Hurt Conversions
Slow Page Load Times: Even a 1-second delay can significantly impact conversion rates. Poor Mobile Experience: Non-responsive design can frustrate mobile users and lead to high bounce rates. Broken Links and 404 Errors: These can interrupt the user journey and negatively impact engagement metrics. Unclear Site Architecture: If users can’t find what they’re looking for, they will likely leave quickly. Unsecured Website (HTTP instead of HTTPS): This can erode trust and discourage conversions, especially on e-commerce sites.Addressing these technical issues improves your site’s performance in search engines and enhances the user experience, leading to better engagement metrics and potentially higher conversions.
Page One Power’s technical SEO audits can help identify and resolve these issues, ensuring your site provides the best possible foundation for user engagement.
The Evolving Link Graph & Strategic Link Building
Google’s approach to evaluating links is evolving. There’s a greater emphasis on the quality and relevance of links rather than sheer quantity.
This shift aligns with the focus on engagement, as high-quality, relevant links are more likely to drive engaged traffic to your site.
How the Link Graph is Changing
Quality Over Quantity: Google is getting better at identifying and discounting low-quality or manipulative links. Relevance Is Key: Links from topically relevant sites carry more weight. User Behavior Matters: Links that drive actual click-throughs and engagement are likely more valuable. Brand Signals: Brand mentions and unlinked citations are gaining importance alongside traditional backlinks.Strategic Link Building for Engagement
Create Linkable Assets: Develop high-quality, original content that naturally attracts links and engages visitors. Focus on Relevant Placements: Seek links from sites in your niche that will likely send interested, engaged traffic. Leverage Brand Building: Increase brand mentions and citations to boost your online presence. Monitor Link Performance: Pay attention to which links drive engaged traffic, not just raw link counts.Page One Power specializes in strategic link building that aligns with these evolving best practices, helping you acquire high-quality, relevant links that boost your search rankings and user engagement.
Conclusion: Adapting to the Engagement-Focused Future
As Google continues to refine its algorithms, websites, and businesses must adapt their strategies accordingly.
By focusing on CRO, addressing technical SEO issues that impact user experience, and evolving your link-building approach, you can create a comprehensive SEO strategy that aligns with Google’s engagement-focused direction.
Remember, the goal is to rank well and provide genuine value to users.
Real-World Results: Case Studies
Consider these case studies:
Kreo, a SaaS company: After implementing keyword-focused content and strategic link-building strategies, Kreo saw impressive results:
216% improvement in total ranking keywords 394% increase in keywords in the top 10 positions 177% increase in year-over-year organic trafficDomotz, a network monitoring software provider: Following a 12-month campaign focused on targeted keyword content and high-quality link acquisition, Domotz experienced:
78% increase in total keyword rankings 18% increase in domain traffic 23% improvement in year-over-year impressionsThese case studies show how our three-pillar approach can improve search visibility and user engagement.
If you need assistance navigating these changes, consider partnering with Page One Power. Our team of experts can provide comprehensive solutions, from technical audits and CRO strategies to high-quality link building, helping you drive sustainable growth.
Introducing SEOntology: The Future Of SEO In The Age Of AI
SEOntology is more than a technical framework. Gain rich insights - learn to standardize SEO data and practices to build a sustainable future with GenAI.
Andrea Volpini
18K Reads
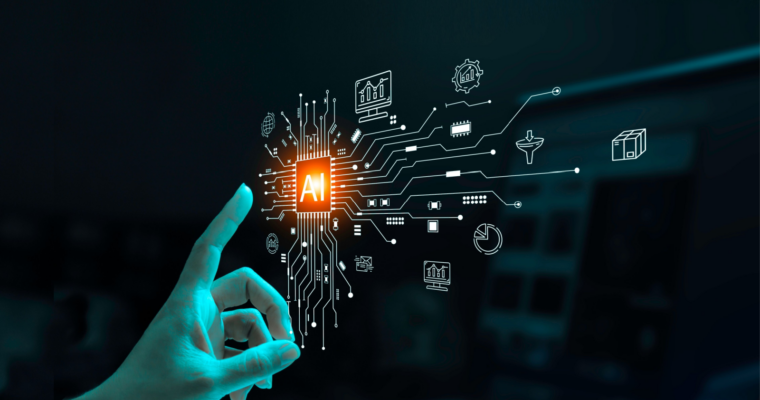
A lot has been said about the remarkable opportunities of Generative AI (GenAI), and some of us have also been extremely vocal about the risks associated with using this transformative technology.
The rise of GenAI presents significant challenges to the quality of information, public discourse, and the general open web. GenAI’s power to predict and personalize content can be easily misused to manipulate what we see and engage with.
How The SEO Industry Can Leverage And Improve Generative AI
Generative AI search engines are contributing to the overall noise, and rather than helping people find the truth and forge unbiased opinions, they tend (at least in their present implementation) to promote efficiency over accuracy, as highlighted by a recent study by Jigsaw, a unit inside Google.
Despite the hype surrounding SEO alligator parties and content goblins, our generation of marketers and SEO professionals has spent years working towards a more positive web environment.
We’ve shifted the marketing focus from manipulating audiences to empowering them with knowledge, ultimately aiding stakeholders in making informed decisions.
Creating an ontology for SEO is a community-led effort that aligns perfectly with our ongoing mission to shape, improve, and provide directions that truly advance human-GenAI interaction while preserving content creators and the Web as a shared resource for knowledge and prosperity.
A Brief Overview Of Traditional SEO Practices And Their Evolution
Traditional SEO practices in the early 2010s focused heavily on keyword optimization. This included tactics like keyword stuffing, link schemes, and creating low-quality content primarily intended for search engines.
Since then, SEO has shifted towards a more user-centric approach. The Hummingbird update (2013) marked Google’s transition towards semantic search, which aims to understand the context and intent behind search queries rather than just the keywords.
This evolution has led SEO pros to focus more on topic clusters and entities than individual keywords, improving content’s ability to answer multiple user queries.
Entities are distinct items like people, places, or things that search engines recognize and understand as individual concepts.
By building content that clearly defines and relates to these entities, organizations can enhance their visibility across various platforms, not just traditional web searches.
This approach ties into the broader concept of entity-based SEO, which ensures that the entity associated with a business is well-defined across the web.
From Static Content To Semantic Data
Fast-forward to today, static content that aims to rank well in search engines is constantly transformed and enriched by semantic data.
This involves structuring information so that it is understandable not only by humans but also by machines.
This transition is crucial for powering Knowledge Graphs and AI-generated responses like those offered by Google’s AIO or Bing Copilot, which provide users with direct answers and links to relevant websites.
Screenshot from Bing Copilot, August 2024
As we move forward, the importance of aligning content with semantic search and entity understanding is growing.
Businesses are encouraged to structure their content in ways that are easily understood and indexed by search engines, thus improving visibility across multiple digital surfaces, such as voice and visual searches.
The use of AI and automation in these processes is increasing, enabling more dynamic interactions with content and personalized user experiences.
Whether we like it or not, AI will help us compare options faster, run deep searches effortlessly, and make transactions without passing through a website.
Automation And AI In SEO
The future of SEO is promising. The SEO service market size is expected to grow from $75.13 billion in 2023 to $88.91 billion in 2024 – a staggering CAGR of 18.3% (according to The Business Research Company) – as it adapts to incorporate reliable AI and semantic technologies.
These innovations support the creation of more dynamic and responsive web environments that adeptly cater to user needs and behaviors.
However, the journey hasn’t been without challenges, especially in large enterprise settings. Implementing AI solutions that are both explainable and strategically aligned with organizational goals has been a complex task.
Building effective AI involves aggregating relevant data and transforming it into actionable knowledge.
This differentiates an organization from competitors using similar language models or development patterns, such as conversational agents or retrieval-augmented generation copilots and enhances its unique value proposition.
Introduction To SEOntology And Its Significance In The Current Digital Landscape
What Is An Ontology For SEO In Layman’s Terms?
Imagine an ontology as a giant instruction manual for describing specific concepts. In the world of SEO, we deal with a lot of jargon, right? Topicality, backlinks, E-E-A-T, structured data – it can get confusing!
An ontology for SEO is a giant agreement on what all those terms mean. It’s like a shared dictionary, but even better. This dictionary doesn’t just define each word. It also shows how they all connect and work together. So, “queries” might be linked to “search intent” and “web pages,” explaining how they all play a role in a successful SEO strategy.
Imagine it as untangling a big knot of SEO practices and terms and turning them into a clear, organized map – that’s the power of ontology!
While Schema.org is a fantastic example of a linked vocabulary, it focuses on defining specific attributes of a web page, like content type or author. It excels at helping search engines understand our content. But what about how we craft links between web pages?
What about the query a web page is most often searched for? These are crucial elements in our day-to-day work, and an ontology can be a shared framework for them as well. Think of it as a playground where everyone is welcome to contribute on GitHub similar to how the Schema.org vocabulary evolves.
The idea of an ontology for SEO is to augment Schema.org with an extension similar to what GS1 did by creating its vocabulary. So, is it a database? A collaboration framework or what? It is all of these things together. SEO ontology operates like a collaborative knowledge base.
It acts as a central hub where everyone can contribute their expertise to define key SEO concepts and how they interrelate. By establishing a shared understanding of these concepts, the SEO community plays a crucial role in shaping the future of human-centered AI experiences.
Screenshot from WebVowl, August 2024SEOntology – a snapshot (see an interactive visualization here).
The Data Interoperability Challenge In The SEO Industry
Let’s start small and review the benefits of a shared ontology with a practical example (here is a slide taken from Emilija Gjorgjevska’s presentation at this year’s ZagrebSEOSummit)
Image from Emilija Gjorgjevska’s, ZagrebSEOSummit, August 2024
Imagine your colleague Valentina uses a Chrome extension to export data from Google Search Console (GSC) into Google Sheets. The data includes columns like “ID,” “Query,” and “Impressions” (as shown on the left). But Valentina collaborates with Jan, who’s building a business layer using the same GSC data. Here’s the problem: Jan uses a different naming convention (“UID,” “Name,” “Impressionen,” and “Klicks”).
Now, scale this scenario up. Imagine working with n different data partners, tools, and team members, all using various languages. The effort to constantly translate and reconcile these different naming conventions becomes a major obstacle to effective data collaboration.
Significant value gets lost in just trying to make everything work together. This is where an SEO ontology comes in. It is a common language, providing a shared name for the same concept across different tools, partners, and languages.
By eliminating the need for constant translation and reconciliation, an SEO ontology streamlines data collaboration and unlocks the true value of your data.
The Genesis Of SEOntology
In the last year, we have witnessed the proliferation of AI Agents and the wide adoption of Retrieval Augmented Generation (RAG) in all its different forms (Modular, Graph RAG, and so on).
RAG represents an important leap forward in AI technology, addressing a key limitation of traditional large language models (LLMs) by letting them access external knowledge.
Traditionally, LLMs are like libraries with one book – limited by their training data. RAG unlocks a vast network of resources, allowing LLMs to provide more comprehensive and accurate responses.
RAGs improve factual accuracy, and context understanding, potentially reducing bias. While promising, RAG faces challenges in data security, accuracy, scalability, and integration, especially in the enterprise sector.
For successful implementation, RAG requires high-quality, structured data that can be easily accessed and scaled.
We’ve been among the first to experiment with AI Agents and RAG powered by the Knowledge Graph in the context of content creation and SEO automation.
Screenshot from Agent WordLift, August 2023
Knowledge Graphs (KGs) Are Indeed Gaining Momentum In RAG Development
Microsoft’s GraphRAG and solutions like LlamaIndex demonstrate this. Baseline RAG struggles to connect information across disparate sources, hindering tasks requiring a holistic understanding of large datasets.
KG-powered RAG approaches like the one offered by LlamaIndex in conjunction with WordLift address this by creating a knowledge graph from website data and using it alongside the LLM to improve response accuracy, particularly for complex questions.
Image from author, August 2024
We have tested workflows with clients in different verticals for over a year.
From keyword research for large editorial teams to the generation of question and answers for ecommerce websites, from content bucketing to drafting the outline of a newsletter or revamping existing articles, we’ve been testing different strategies and learned a few things along the way:
1. RAG Is Overhyped
It is simply one of many development patterns that achieve a goal of higher complexity. A RAG (or Graph RAG) is meant to help you save time finding an answer. It’s brilliant but doesn’t solve any marketing tasks a team must handle daily. You need to focus on the data and the data model.
While there are good RAGs and bad RAGs, the key differentiation is often represented by the “R” part of the equation: the Retrieval. Primarily, the retrieval differentiates a fancy demo from a real-world application, and behind a good RAG, there is always good data. Data, though, is not just any type of data (or graph data).
It is built around a coherent data model that makes sense for your use case. If you build a search engine for wines, you need to get the best dataset and model the data around the features a user will rely on when looking for information.
So, data is important, but the data model is even more important. If you are building an AI Agent that has to do things in your marketing ecosystem, you must model the data accordingly. You want to represent the essence of web pages and content assets.
Image from author, August 2024
2. Not Everyone Is Great At Prompting
Expressing a task in written form is hard. Prompt engineering is going at full speed towards automation (here is my article on going from prompting to prompt programming for SEO) as only a few experts can write the prompt that brings us to the expected outcome.
This poses several challenges for the design of the user experience of autonomous agents. Jakon Nielsen has been very vocal about the negative impact of prompting on the usability of AI applications:
“One major usability downside is that users must be highly articulate to write the required prose text for the prompts.”
Even in rich Western countries, statistics provided by Nielsen tell us that only 10% of the population can fully utilize AI!
Simple Prompt Using Chain-of-Thought (CoT) | More Sophisticated Prompt Combining Graph-of-Thought (GoT) and Chain-of-Knowledge (CoK) |
“Explain step-by-step how to calculate the area of a circle with a radius of 5 units.” | “Using the Graph-of-Thought (GoT) and Chain-of-Knowledge (CoK) techniques, provide a comprehensive explanation of how to calculate the area of a circle with a radius of 5 units. Your response should: Start with a GoT diagram that visually represents the key concepts and their relationships, including: Circle Radius Area Pi (π) Formula for circle area Follow the GoT diagram with a CoK breakdown that: a) Defines each concept in the diagram b) Explains the relationships between these concepts c) Provides the historical context for the development of the circle area formula Present a step-by-step calculation process, including: a) Stating the formula for the area of a circle b) Explaining the role of each component in the formula c) Showing the substitution of values d) Performing the calculation e) Rounding the result to an appropriate number of decimal places Conclude with practical applications of this calculation in real-world scenarios. Throughout your explanation, ensure that each step logically follows the previous one, creating a clear chain of reasoning from basic concepts to the final result.” This improved prompt incorporates GoT by requesting a visual representation of the concepts and their relationships. It also employs CoK by asking for definitions, historical context, and connections between ideas. The step-by-step breakdown and real-world applications further enhance the depth and practicality of the explanation.” |
3. You Shall Build Workflows To Guide The User
The lesson learned is that we must build detailed standard operating procedures (SOP) and written protocols that outline the steps and processes to ensure consistency, quality, and efficiency in executing particular optimization tasks.
We can see empirical evidence of the rise of prompt libraries like the one offered to users of Anthropic models or the incredible success of projects like AIPRM.
In reality, we learned that what creates business value is a series of ci steps that help the user translate the context he/she is navigating in into a consistent task definition.
We can start to envision marketing tasks like conducting keyword research as a Standard Operating Procedure that can guide the user across multiple steps (here is how we intend the SOP for keyword discovery using Agent WordLift)
4. The Great Shift To Just-in-Time UX
In traditional UX design, information is pre-determined and can be organized in hierarchies, taxonomies, and pre-defined UI patterns. As AI becomes the interface to the complex world of information, we’re witnessing a paradigm shift.
UI topologies tend to disappear, and the interaction between humans and AI remains predominantly dialogic. Just-in-time assisted workflows can help the user contextualize and improve a workflow.
You need to think in terms of business value creation, focus on the user’s interactive journey, and facilitate the interaction by creating a UX on the fly. Taxonomies remain a strategic asset, but they operate behind the scenes as the user is teleported from one task to another, as recently brilliantly described by Yannis Paniaras from Microsoft.Image from “The Shift to Just-In-Time UX: How AI is Reshaping User Experiences” by Yannis Paniaras, August 2024
5. From Agents To RAG (And GraphRAG) To Reporting
Because the user needs a business impact and RAG is only part of the solution, the focus quickly shifts from more generic questions and answering user patterns to advanced multi-step workflows.
The biggest issue, though, is what outcome the user needs. If we increase the complexity to capture the highest business goals, it is not enough to, let’s say, “query your data” or “chat with your website.”
A client wants a report, for example, of what is the thematic consistency of content within the entire website (this is a concept that we recently discovered as SiteRadus in Google’s massive data leak), the overview of the seasonal trends across hundreds of paid campaigns, or the ultimate review of the optimization opportunities related to the optimization of Google Merchant Feed.
You must understand how the business operates and what deliverables you will pay for. What concrete actions could boost the business? What questions need to be answered?
This is the start of creating a tremendous AI-assisted reporting tool.
How Can A Knowledge Graph (KG) Be Coupled With An Ontology For AI Alignment, Long-term Memory, And Content Validation?
The three guiding principles behind SEOntology:
Making SEO data interoperable to facilitate the creation of knowledge graphs while reducing unneeded crawls and vendor locked-in; Infusing SEO know-how into AI agents using a domain-specific language. Collaboratively sharing knowledge and tactics to improve findability and prevent misuse of Generative AI.When you deal with at least two data sources in your SEO automation task, you will already see the advantage of using SEOntology.
SEOntology As “The USB-C Of SEO/Crawling Data”
Standardizing data about content assets, products, user search behavior, and SEO insights is strategic. The goal is to have a “shared representation” of the Web as a communication channel.
Let’s take a step backward. How does a Search Engine represent a web page? This is our starting point here. Can we standardize how a crawler would represent data extracted from a website? What are the advantages of adopting standards?
Practical Use Cases
Integration With Botify And Dynamic Internal Linking
Over the past few months, we’ve been working closely with the Botify team to create something exciting: a Knowledge Graph powered by Botify’s crawl data and enhanced by SEOntology. This collaboration is opening up new possibilities for SEO automation and optimization.
Leveraging Existing Data With SEOntology
Here’s the cool part: If you’re already using Botify, we can tap into that goldmine of data you’ve collected. No need for additional crawls or extra work on your part. We use the Botify Query Language (BQL) to extract and transform the needed data using SEOntology.
Think of SEOntology as a universal translator for SEO data. It takes the complex information from Botify and turns it into a format that’s not just machine-readable but machine-understandable. This allows us to create a rich, interconnected Knowledge Graph filled with valuable SEO insights.
What This Means for You
Once we have this Knowledge Graph, we can do some pretty amazing things:
Automated Structured Data: We can automatically generate structured data markup for your product listing pages (PLPs). This helps search engines better understand your content, potentially improving your visibility in search results. Dynamic Internal Linking: This is where things get really interesting. We use the data in the Knowledge Graph to create smart, dynamic internal links across your site. Let me break down how this works and why it’s so powerful.In the diagram below, we can also see how data from Botify can be blended with data from Google Search Console.
While in most implementations, Botify already imports this data into its crawl projects, when this is not the case, we can trigger a new API request and import clicks, impressions, and positions from GSC into the graph.
Collaboration With Advertools For Data Interoperability
Similarly, we collaborated with the brilliant Elias Dabbas, creator of Advertools — a favorite Python library among marketers – to automate a wide range of marketing tasks.
Our joint efforts aim to enhance data interoperability, allowing for seamless integration and data exchange across different platforms and tools.
In the first Notebook, available in the SEOntology GitHub repository, Elias showcases how we can effortlessly construct attributes for the WebPage class, including title, meta description, images, and links. This foundation enables us to easily model complex elements, such as internal linking strategies. See here the structure:
Internal_Links anchorTextContent NoFollow LinkWe can also add a flag if the page is already using schema markup:
usesSchemaFormalizing What We Learned From The Analysis Of The Leaked Google Search Documents
While we want to be extremely conscious in deriving tactics or small schemes from Google’s massive leak, and we are well aware that Google will quickly prevent any potential misuse of such information, there is a great level of information that, based on what we learned, can be used to improve how we represent web content and organize marketing data.
Despite these constraints, the leak offers valuable insights into improving web content representation and marketing data organization. To democratize access to these insights, I’ve developed a Google Leak Reporting tool designed to make this information readily available to SEO pros and digital marketers.
For instance, understanding Google’s classification system and its segmentation of websites into various taxonomies has been particularly enlightening. These taxonomies – such as ‘verticals4’, ‘geo’, and ‘products_services’ – play a crucial role in search ranking and relevance, each with unique attributes that influence how websites and content are perceived and ranked in search results.
By leveraging SEOntology, we can adopt some of these attributes to enhance website representation.
Now, pause for a second and imagine transforming the complex SEO data you manage daily through tools like Moz, Ahrefs, Screaming Frog, Semrush, and many others into an interactive graph. Now, envision an Autonomous AI Agent, such as Agent WordLift, at your side.
This agent employs neuro-symbolic AI, a cutting-edge approach that combines neural learning capabilities with symbolic reasoning, to automate SEO tasks like creating and updating internal links. This streamlines your workflow and introduces a level of precision and efficiency previously unattainable.
SEOntology serves as the backbone for this vision, providing a structured framework that enables the seamless exchange and reuse of SEO data across different platforms and tools. By standardizing how SEO data is represented and interconnected, SEOntology ensures that valuable insights derived from one tool can be easily applied and leveraged by others. For instance, data on keyword performance from SEMrush could inform content optimization strategies in WordLift, all within a unified, interoperable environment. This not only maximizes the utility of existing data but also accelerates the automation and optimization processes that are crucial for effective marketing.
Infusing SEO Know-How Into AI Agents
As we develop a new agentic approach to SEO and digital marketing, SEOntology serves as our domain-specific language (DSL) for encoding SEO skills into AI agents. Let’s look at a practical example of how this works.
Screenshot from WordLift, August 2024
We’ve developed a system that makes AI agents aware of a website’s organic search performance, enabling a new kind of interaction between SEO professionals and AI. Here’s how the prototype works:
System Components
Knowledge Graph: Stores Google Search Console (GSC) data, encoded with SEOntology. LLM: Translates natural language queries into GraphQL and analyzes data. AI Agent: Provides insights based on the analyzed data.Human-Agent Interaction
Image from author, August 2024
The diagram illustrates the flow of a typical interaction. Here’s what makes this approach powerful:
Natural Language Interface: SEO professionals can ask questions in plain language without constructing complex queries. Contextual Understanding: The LLM understands SEO concepts, allowing for more nuanced queries and responses. Insightful Analysis: The AI agent doesn’t just retrieve data; it provides actionable insights, such as: Identifying top-performing keywords. Highlighting significant performance changes. Suggesting optimization opportunities. Interactive Exploration: Users can ask follow-up questions, enabling a dynamic exploration of SEO performance.By encoding SEO knowledge through SEOntology and integrating performance data, we’re creating AI agents that can provide context-aware, nuanced assistance in SEO tasks. This approach bridges the gap between raw data and actionable insights, making advanced SEO analysis more accessible to professionals at all levels.
This example illustrates how an ontology like SEOntology can empower us to build agentic SEO tools that automate complex tasks while maintaining human oversight and ensuring quality outcomes. It’s a glimpse into the future of SEO, where AI augments human expertise rather than replacing it.
Human-In-The-Loop (HTIL) And Collaborative Knowledge Sharing
Let’s be crystal clear: While AI is revolutionizing SEO and Search, humans are the beating heart of our industry. As we dive deeper into the world of SEOntology and AI-assisted workflows, it’s crucial to understand that Human-in-the-Loop (HITL) isn’t just a fancy add-on—it’s the foundation of everything we’re building.
The essence of creating SEOntology is to transfer our collective SEO expertise to machines while ensuring we, as humans, remain firmly in the driver’s seat. It’s not about handing over the keys to AI; it’s about teaching it to be the ultimate co-pilot in our SEO journey.
Human-Led AI: The Irreplaceable Human Element
SEOntology is more than a technical framework – it’s a catalyst for collaborative knowledge sharing that emphasizes human potential in SEO. Our commitment extends beyond code and algorithms to nurturing skills and expanding the capabilities of new-gen marketers and SEO pros.
Why? Because AI’s true power in SEO is unlocked by human insight, diverse perspectives, and real-world experience. After years of working with AI workflows, I’ve realized that agentive SEO is fundamentally human-centric. We’re not replacing expertise; we’re amplifying it.
We deliver more efficient and trustworthy results by blending cutting-edge tech with human creativity, intuition, and ethical judgment. This approach builds trust with clients within our industry and across the web.
Here’s where humans remain irreplaceable:
Understanding Business Needs: AI can crunch numbers but can’t replace the nuanced understanding of business objectives that seasoned SEO professionals bring. We need experts who can translate client goals into actionable SEO strategies. Identifying Client Constraints: Every business is unique, with its limitations and opportunities. It takes human insight to navigate these constraints and develop tailored SEO approaches that work within real-world parameters. Developing Cutting-Edge Algorithms: The algorithms powering our AI tools don’t materialize out of thin air. We need brilliant minds to develop state-of-the-art algorithms, learn from human input, and continually improve. Engineering Robust Systems: Behind every smooth-running AI tool is a team of software engineers who ensure our systems are fast, secure, and reliable. This human expertise keeps our AI assistants running like well-oiled machines. Passion for a Better Web: At the heart of SEO is a commitment to making the web a better place. We need people who share Tim Berners’s—Lee’s vision—people who are passionate about developing the web of data and improving the digital ecosystem for everyone. Community Alignment and Resilience: We need to unite to analyze the behavior of search giants and develop resilient strategies. It’s about solving our problems innovatively as individuals and as a collective force. This is what I always loved about the SEO industry!Extending The Reach Of SEOntology
As we continue to develop SEOntology, we’re not operating in isolation. Instead, we’re building upon and extending existing standards, particularly Schema.org, and following the successful model of the GS1 Web Vocabulary.
SEOntology As An Extension Of Schema.org
Schema.org has become the de facto standard for structured data on the web, providing a shared vocabulary that webmasters can use to markup their pages.
However, while Schema.org covers a broad range of concepts, it doesn’t delve deeply into SEO-specific elements. This is where SEOntology comes in.
An extension of Schema.org, like SEOntology, is essentially a complementary vocabulary that adds new types, properties, and relationships to the core Schema.org vocabulary.
This allows us to maintain compatibility with existing Schema.org implementations while introducing SEO-specific concepts not covered in the core vocabulary.
Learning From GS1 Web Vocabulary
The GS1 Web Vocabulary offers a great model for creating a successful extension that interacts seamlessly with Schema.org. GS1, a global organization that develops and maintains supply chain standards, created its Web Vocabulary to extend Schema.org for e-commerce and product information use cases.
The GS1 Web Vocabulary demonstrates, even recently, how industry-specific extensions can influence and interact with schema markup:
Real-world impact: The https://schema.org/Certification property, now officially embraced by Google, originated from GS1’s https://www.gs1.org/voc/CertificationDetails. This showcases how extensions can drive the evolution of Schema.org and search engine capabilities.We want to follow a similar approach to extend Schema.org and become the standard vocabulary for SEO-related applications, potentially influencing future search engine capabilities, AI-driven workflows, and SEO practices.
Much like GS1 defined their namespace (gs1:) while referencing schema terms, we have defined our namespace (seovoc:) and are integrating the classes within the Schema.org hierarchy when possible.
The Future Of SEOntology
SEOntology is more than just a theoretical framework; it’s a practical tool designed to empower SEO professionals and tool makers in an increasingly AI-driven ecosystem.
Here’s how you can engage with and benefit from SEOntology.
If you’re developing SEO tools:
Data Interoperability: Implement SEOntology to export and import data in a standardized format. This ensures your tools can easily interact with other SEOntology-compliant systems. AI-Ready Data: By structuring your data according to SEOntology, you’re making it more accessible for AI-driven automations and analyses.If you’re an SEO professional:
Contribute to Development: Just like with Schema.org, you can contribute to SEOntology’s evolution. Visit its GitHub repository to: Raise issues for new concepts or properties you think should be included. Propose changes to existing definitions. Participate in discussions about the future direction of SEOntology. Implement in Your Work: Start using SEOntology concepts in your structured data.In Open Source We Trust
SEOntology is an open-source effort, following in the footsteps of successful projects like Schema.org and other shared linked vocabularies.
All discussions and decisions will be public, ensuring the community has a say in SEOntology’s direction. As we gain traction, we’ll establish a committee to steer its development and share regular updates.
Conclusion And Future Work
The future of marketing is human-led, not AI-replaced. SEOntology isn’t just another buzzword – it’s a step towards this future. SEO is strategic for the development of agentive marketing practices.
SEO is no longer about rankings; it’s about creating intelligent, adaptive content and fruitful dialogues with our stakeholders across various channels. Standardizing SEO data and practices is strategic to build a sustainable future and to invest in responsible AI.
Are you ready to join this revolution?
There are three guiding principles behind the work of SEOntology that we need to make clear to the reader:
As AI needs semantic data, we need to make SEO data interoperable, facilitating the creation of knowledge graphs for everyone. SEOntology is the USB-C of SEO/crawling data. Standardizing data about content assets and products and how people find content, products, and information in general is important. This is the first objective. Here, we have two practical use cases. We have a connector for WordLift that gets crawl data from the Botify crawler and helps you jump-start a KG that uses SEOntology as a data model. We are also working with Advertools, an open-source crawler and SEO tool, to make data interoperable with SEOntology; As we progress with the development of a new agentic way of doing SEO and digital marketing, we want to infuse the know-how of SEO using SEOntology, a domain-specific language to infuse the SEO mindset to SEO agents (or multi-agent systems like Agent WordLift). In this context, the skill required to create dynamic internal links is encoded as nodes in a knowledge graph, and opportunities become triggers to activate workflows. We expect to work with human-in-the-loop HITL, meaning that the ontology will become a way to collaboratively share knowledge and tactics that help improve findability and prevent the misuse of Generative AI that is polluting the Web today.Project Overview
Public repository Thread on XThis work on SEOntology is the product of collaboration. I extend my sincere thanks to the WordLift team, especially CTO David Riccitelli. I also appreciate our clients for their dedication to innovation in SEO through knowledge graphs. Special thanks to Milos Jovanovik and Emilia Gjorgjevska for their critical expertise. Lastly, I’m grateful to the SEO community and the SEJ editorial team for their support in sharing this work.
More resources:
AI Has Changed How Search Works Leveraging Generative AI Tools For SEO How Search Engines WorkFeatured Image: tech_BG/Shutterstock
How To Use Python To Test SEO Theories (And Why You Should)
Explore the steps involved in using Python to test SEO theories and ensure the success of your SEO implementation.
Andreas Voniatis
5.9K Reads
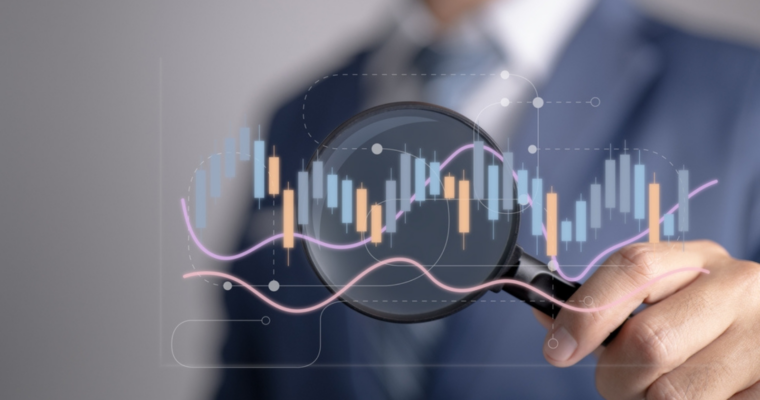
When working on sites with traffic, there is as much to lose as there is to gain from implementing SEO recommendations.
The downside risk of an SEO implementation gone wrong can be mitigated using machine learning models to pre-test search engine rank factors.
Pre-testing aside, split testing is the most reliable way to validate SEO theories before making the call to roll out the implementation sitewide or not.
We will go through the steps required on how you would use Python to test your SEO theories.
Choose Rank Positions
One of the challenges of testing SEO theories is the large sample sizes required to make the test conclusions statistically valid.
Split tests – popularized by Will Critchlow of SearchPilot – favor traffic-based metrics such as clicks, which is fine if your company is enterprise-level or has copious traffic.
If your site doesn’t have that envious luxury, then traffic as an outcome metric is likely to be a relatively rare event, which means your experiments will take too long to run and test.
Instead, consider rank positions. Quite often, for small- to mid-size companies looking to grow, their pages will often rank for target keywords that don’t yet rank high enough to get traffic.
Over the timeframe of your test, for each data point of time, for example day, week or month, there are likely to be multiple rank position data points for multiple keywords. In comparison to using a metric of traffic (which is likely to have much less data per page per date), which reduces the time period required to reach a minimum sample size if using rank position.
Thus, rank position is great for non-enterprise-sized clients looking to conduct SEO split tests who can attain insights much faster.
Google Search Console Is Your Friend
Deciding to use rank positions in Google makes using the data source a straightforward (and conveniently a low-cost) decision in Google Search Console (GSC), assuming it’s set up.
GSC is a good fit here because it has an API that allows you to extract thousands of data points over time and filter for URL strings.
While the data may not be the gospel truth, it will at least be consistent, which is good enough.
Filling In Missing Data
GSC only reports data for URLs that have pages, so you’ll need to create rows for dates and fill in the missing data.
The Python functions used would be a combination of merge() (think VLOOKUP function in Excel) used to add missing data rows per URL and filling the data you want to be inputed for those missing dates on those URLs.
For traffic metrics, that’ll be zero, whereas for rank positions, that’ll be either the median (if you’re going to assume the URL was ranking when no impressions were generated) or 100 (to assume it wasn’t ranking).
The code is given here.
Check The Distribution And Select Model
The distribution of any data represents its nature, in terms of where the most popular value (mode) for a given metric, say rank position (in our case the chosen metric) is for a given sample population.
The distribution will also tell us how close the rest of the data points are to the middle (mean or median), i.e., how spread out (or distributed) the rank positions are in the dataset.
This is critical as it will affect the choice of model when evaluating your SEO theory test.
Using Python, this can be done both visually and analytically; visually by executing this code:
Image from author, July 2024
The chart above shows that the distribution is positively skewed (think skewer pointing right), meaning most of the keywords rank in the higher-ranked positions (shown towards the left of the red median line). To run this code please make sure to install required libraries via command pip install pandas plotnine:
Now, we know which test statistic to use to discern whether the SEO theory is worth pursuing. In this case, there is a selection of models appropriate for this type of distribution.
Minimum Sample Size
The selected model can also be used to determine the minimum sample size required.
The required minimum sample size ensures that any observed differences between groups (if any) are real and not random luck.
That is, the difference as a result of your SEO experiment or hypothesis is statistically significant, and the probability of the test correctly reporting the difference is high (known as power).
This would be achieved by simulating a number of random distributions fitting the above pattern for both test and control and taking tests.
The code is given here.
When running the code, we see the following:
To break it down, the numbers represent the following using the example below:
(39.333,: proportion of simulation runs or experiments in which significance will be reached, i.e., consistency of reaching significance and robustness.
1.0) : statistical power, the probability the test correctly rejects the null hypothesis, i.e., the experiment is designed in such a way that a difference will be correctly detected at this sample size level.
60000: sample size
The above is interesting and potentially confusing to non-statisticians. On the one hand, it suggests that we’ll need 230,000 data points (made of rank data points during a time period) to have a 92% chance of observing SEO experiments that reach statistical significance. Yet, on the other hand with 10,000 data points, we’ll reach statistical significance – so, what should we do?
Experience has taught me that you can reach significance prematurely, so you’ll want to aim for a sample size that’s likely to hold at least 90% of the time – 220,000 data points are what we’ll need.
This is a really important point because having trained a few enterprise SEO teams, all of them complained of conducting conclusive tests that didn’t produce the desired results when rolling out the winning test changes.
Hence, the above process will avoid all that heartache, wasted time, resources and injured credibility from not knowing the minimum sample size and stopping tests too early.
Assign And Implement
With that in mind, we can now start assigning URLs between test and control to test our SEO theory.
In Python, we’d use the np.where() function (think advanced IF function in Excel), where we have several options to partition our subjects, either on string URL pattern, content type, keywords in title, or other depending on the SEO theory you’re looking to validate.
Use the Python code given here.
Strictly speaking, you would run this to collect data going forward as part of a new experiment. But you could test your theory retrospectively, assuming that there were no other changes that could interact with the hypothesis and change the validity of the test.
Something to keep in mind, as that’s a bit of an assumption!
Test
Once the data has been collected, or you’re confident you have the historical data, then you’re ready to run the test.
In our rank position case, we will likely use a model like the Mann-Whitney test due to its distributive properties.
However, if you’re using another metric, such as clicks, which is poisson-distributed, for example, then you’ll need another statistical model entirely.
The code to run the test is given here.
Once run, you can print the output of the test results:
The above is the output of an experiment I ran, which showed the impact of commercial landing pages with supporting blog guides internally linking to the former versus unsupported landing pages.
In this case, we showed that offer pages supported by content marketing enjoy a higher Google rank by 17 positions (22.58 – 5.87) on average. The difference is significant, too, at 98%!
However, we need more time to get more data – in this case, another 210,000 data points. As with the current sample size, we can only be sure that <10% of the time, the SEO theory is reproducible.
Split Testing Can Demonstrate Skills, Knowledge And Experience
In this article, we walked through the process of testing your SEO hypotheses, covering the thinking and data requirements to conduct a valid SEO test.
By now, you may come to appreciate there is much to unpack and consider when designing, running and evaluating SEO tests. My Data Science for SEO video course goes much deeper (with more code) on the science of SEO tests, including split A/A and split A/B.
As SEO professionals, we may take certain knowledge for granted, such as the impact content marketing has on SEO performance.
Clients, on the other hand, will often challenge our knowledge, so split test methods can be most handy in demonstrating your SEO skills, knowledge, and experience!
More resources:
Using Python To Explain Homepage Redirection To C-Suite (Or Any SEO Best Practise) What Data Science Can Do for Site Architectures An Introduction To Python & Machine Learning For Technical SEOFeatured Image: UnderhilStudio/Shutterstock
3 Types Of PR & SEO Funnels That Will Maximize Conversions
Discover how integrating digital PR with SEO can drive qualified leads and ensure consistent messaging to guide customers to website engagement.
Kevin Rowe
5.1K Reads
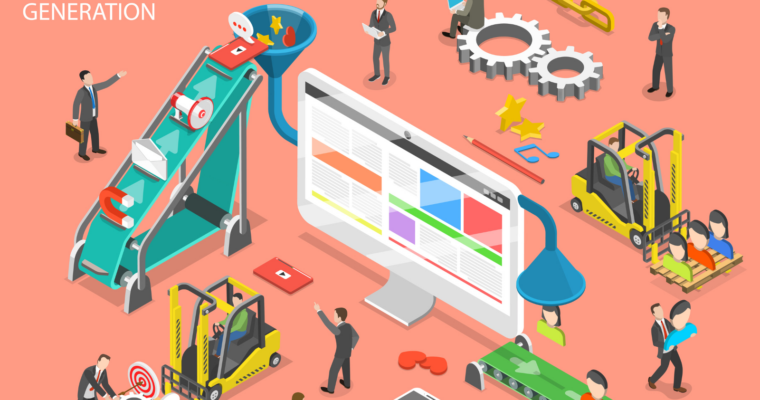
Aligning your content with media coverage can drive high conversion rates because it influences search behavior and creates a funnel from media to search and through the website.
I’ve seen the best conversion rates and most qualified leads from SEO and content marketing when I coupled it with a digital PR strategy, creating these funnels.
Are SEO and content marketing part of your marketing mix? Do you see low conversion rates? Is your SEO program just failing to achieve business results or simple conversions?
Then, these three ways to optimize funnels can change the success trajectory of your entire program by creating a PR & SEO funnel.
Article overview:
Integrated digital PR and SEO: Combining digital PR with SEO boosts conversion rates by ensuring consistent messaging that guides customers from media exposure to website engagement and conversion. Funnel optimization strategies: I describe three methods to enhance SEO through content: 1.) Branded reports that attract media coverage and drive web traffic. 2.) Guides in new market categories to dominate search rankings and establish market leadership. 3.) Feature articles that trigger brand searches and optimize homepage messaging to convert traffic. Success case studies: This article will highlight effective examples like HubSpot’s annual marketing report and Drift’s conversational marketing platform, showing how targeted content and strategic media coverage can significantly increase traffic and conversions. I also mention Sweetgreen’s strategic use of media stories to align with homepage updates that improve brand search funnels.Before diving into case studies, it’s important to understand why and how digital PR & SEO work together.
Why Create A PR & SEO Funnel
Marq’s Brand Consistency report found that 68% of businesses said brand message consistency contributed to revenue growth by 10% to 20% or more.
Image created by author, May 2024
Optimizing content for digital PR creates consistent messaging to nudge the audience from the media, through search engines, to specific web pages, and finally to conversion.
The media is at the top of the funnel, and you use media to drive demand and interest in an idea.
For example, create an industry trend report that identifies an opportunity or a major pain point for an audience. Media loves data, so use these reports to gain media coverage.
The coverage then drives searches for keywords your website owns. These can be the company or product brand name, a new concept, or just keywords you dominate for.
Keywords you own create a much higher click-through rate (CTR) than other keywords.
For brand keywords, a site can get up to a 60% CTR. An audience searching for these keywords tends to be more willing to submit an email to get a document or contact.
Image from Backlinko, May 2024
Instead of telling you how to optimize content for these funnels, let’s look at some examples.
These are three ways to optimize content that will change the way you do SEO.
1. Branded Reports For Data Shares
Create a funnel from your data by optimizing the report’s name and using it in a digital PR data campaign.
This approach creates a funnel from media through search engines to website conversion by owning your “state of industry” report or creating a study with its own brand name.
The audience will often read about the report in the media, on a blog, or hear about it referenced on a podcast, then search for it in Google or click the report link.
HubSpot and Conductor create two reports that garner some attention.
HubSpot’s State Of Marketing
HubSpot creates a “State of Marketing” report each year that gains a significant number of links and brand mentions.
The report covers a wide range of topics and trends in marketing that can impact marketers’ decisions, ideally affecting everything from how to use AI to which social channels produce the best ROI.
Screenshot by author, May 2024
According to Semrush, the report URL generated links from 7,400 referring domains. A quick Google search for the search operator “hubspot” and “state of marketing” –hubspot.com uncovered a significant number of brand mentions in the report.
Brand mentions of the report names can drive the audience to search for the report on Google and then download it from the website. This is a simple but effective funnel.
Screenshot by author, May 2024
Conductor’s State Of SEO
Conductor, an enterprise SEO software, has a “State of SEO” report that surveyed over 400 digital marketing, SEO, and content marketing experts to identify actionable insights.
Screenshot by author, May 2024
Conductor’s report received a brand mention and a direct link on entrepreneur.com, which can create a click or brand search.
Screenshot by author May 2024
Branded studies are one of my favorite funnels, as the reports can be named and focused on a specific persona or audience segment.
2. Guides In A New Category
This is by far my favorite approach to integrated digital PR & SEO because it can make competition irrelevant.
“Category design” is a strategy that involves creating and leading your own new market category or strategy. This approach involves creating foundational training content to help your audience see and capitalize on the value of your approach.
The foundational content should rank in search engines for the category name, then use digital PR to drive demand and interest in the category.
Drift is a wildly successful example of this strategy.
Conversational Marketing By Drift
I use the Drift example of category design whenever I’m involved in a planning session to identify or design a new category.
Drift is essentially a chatbot. However, the product is marketed as a “conversational marketing” platform. Functionally, it tailors a chat experience on a website based on the stage of the buyer’s journey and qualifies the leads.
Drift created the category “conversational marketing” and positioned the company as a platform for a conversational marketing platform, as you can see from its main navigation.
Screenshot by author, May 2024
Drift was founded in 2015 and, shortly after, started developing the “conversational marketing” category. A few years after the company’s launch, the audience started searching Google for the concept.
Screenshot from Google Trends, May 2024
Drift is still highly visible in Google for the term, ranking in the “What is Conversational Marketing” guide.
The launch of conversational marketing was so well executed that even HubSpot followed along and created a beginner’s guide, ranking just below Drift.
Screenshot from search for [conversational marketing], May 2024
If you’re creating a new category or trying to drive brand search for your company, create customer story funnels.
3. Homepage For Feature Articles
Feature articles can drive brand search spikes, and optimizing the homepage messaging can move the audience further down the funnel to take action on a website.
Sweetgreen, the fast-casual restaurant chain that serves salads, is a great example.
In early May, the New York Times and other publications published articles about Sweetgreen starting to offer steak. The angle was that Sweetgreen’s mission “committed to becoming carbon neutral by 2027” was at “steak” because the meat industry is a major producer of carbon.
Screenshot by author, May 2024
The articles about Sweetgreen’s addition of steak to the menu caused Google Trends to show a significant 36% spike in search for the brand name around May 7th.
Screenshot from Google Trends, May 2024
When a given audience searches for a brand name, most will end up on the homepage. Have you ever noticed a spike in traffic and higher conversions from the homepage? Very often, this is a brand search.
Sweetgreen updated its homepage banner to show steak as the first image. This creates a cohesive messaging funnel for the audience, so they will either convert when they enter the site or improve their recollection of the new steak option.
Screenshot by author,May 2024
Securing feature articles that align with the homepage messaging creates a powerful funnel as the audience searches the brand in search engines.
Start Optimizing
Digital PR with SEO can transform content marketing strategies, leading to enhanced visibility, higher traffic, and increased conversion rates.
A website can significantly influence audience behavior by aligning content with media coverage through branded reports, innovative category guides, and strategically placed feature articles.
The provided case studies underline the potential of these strategies to attract attention and drive meaningful engagement and growth.
More resources:
How A Full-Funnel SEO & PR Strategy Can Drive Leads & Sales How To Use SEO To Target Your Audience Throughout The Funnel The Dark Side Of Link BuildingFeatured Image: TarikVision/Shutterstock
How To Think About SEO, Content & PR Measurement (Indicated In The Google Leak)
Get ahead in SEO with PR engagement metrics. Learn how to evaluate the impact of integrated campaigns and influence audience search behavior.
Kevin Rowe
3.6K Reads
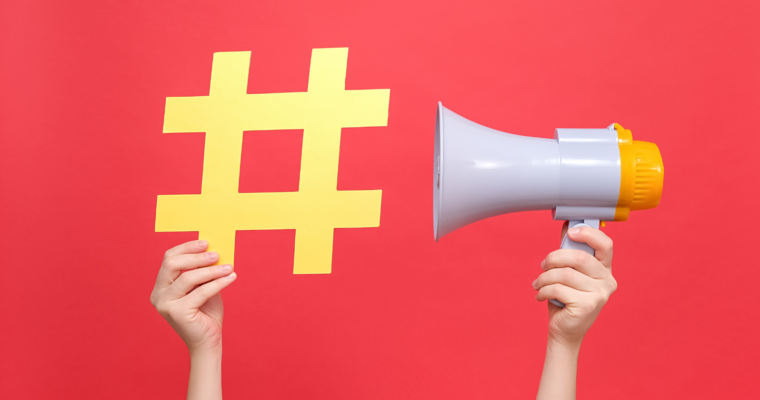
Google’s recent leak highlighted engagement as part of the ranking system, alluding to the importance of influencing audience behavior to drive SEO-specific metrics, like ranking or organic visibility.
That said, I’ve been using simple variations of these measures for a while to evaluate the impact of integrated PR and SEO campaigns. I don’t think the idea of measuring search behavior is new, but the Google leaks shed some light on its importance.
For sustainable growth in organic visibility and rankings, SEO strategies need to pivot to include measures that reflect how strongly owned assets, marketing assets, and messaging influence an audience’s search behavior.
Google’s broader objective to rank content that is genuinely helpful to specific audience segments is an important context for considering this shift.
So, SEO pros should evaluate website performance based on engagement-driven metrics like asset NPS, idea adoption rate, and time to activation, which will be important for directly and indirectly maximizing organic search visibility.
Why Measure Influence
The recent Google leaks highlight the growing importance of audience engagement measures in ranking pages.
This highlights the importance of integrating SEO, content creation, and PR, where influencing audience behavior becomes a key measure.
I see it like this:
Google emphasizes engagement: The Google leaks suggest that Google places a lot of weight on user engagement measures such as click data, repeat visitors, site traffic, or related click data. Despite being incomplete and likely outdated information, it is one of many examples of Google using user engagement in some way. AI integration into the algorithm: With AI being integrated more heavily into Google’s ranking systems, AI could interpret and use this user engagement data to influence ranking. Brand search: Site traffic from brand search is an indicator of audience engagement and can influence organic visibility.But to drive audience engagement, we have to think beyond simple SEO activities like link building, creating keyword-focused content, or technical SEO.
The future of search marketing is designing scenarios that influence an audience’s search behavior.
Ideal Search Behavior Scenario
The audience’s journey is more complex today than ever because they use many different sources to learn about their problems, the solutions, and the opportunities they create. However, this scenario simplifies how to think about your search strategy.
Scenario: You create an asset, you get PR coverage, and the audience searches the asset in Google (maybe they don’t find it based on keywords, then search your brand name). Then, they keep returning to your site for new assets or resources to solve their problems or create an opportunity (the original one as a resource or for your offering).
Simple Search Behavior Scenario Statement:
I need to create a content asset about [a problem or opportunity], to get coverage about [an asset of the asset] that the audience will prompt because [audience interest], which will drive my audience to search for [category or terms you own], and they will immediately or return to the site to take action because [solve a problem or create an opportunity].
You’ll have to modify this based on your specific website event goals, but the statement’s essence will guide you in the right direction.
This direction will allow you to focus on the much more significant but more difficult-to-impact measures below.
I have a foundation in product management and marketing, so I adopted these measures from product marketing concepts since they directly relate to audience actions.
Measure 1: Asset NPS
How likely is your audience to promote your content assets or ideas?
NPS score is used to gauge an audience’s loyalty and satisfaction using a survey question: “How likely are you to recommend our content to a friend or colleague?”
Respondents can provide a rating from 0-10.
Promoters (9-10): Loyal and enthusiastic audience who keep talking about and referring your content or ideas to others. Passives (7-8): Satisfied with content but not an overly enthusiastic audience who will listen to a competitor’s point of view. Detractors (0-6): Unhappy audience that speaks negatively about your content.High NPS indicates strong audience engagement, boosting engagement, and can indirectly influence organic visibility.
Typically, you’d have to survey an audience to gather the data. Use Google Forms, Survey Monkey, or any survey tool with a rating scale to collect questions.
Pro tip: Survey the audience on your site, the following you have on social media, or the email list you’re building as a result of the audience submitting contact info on the site or even through a newsletter.
Measure 2: Idea Adoption Rate
Does your audience adopt your ideas?
The adoption rate of an idea refers to the percentage of the audience segment that starts using the idea after you launch the asset.
This is a key measure to understand if your audience is accepting a particular idea, providing insights into engagement and market fit. This could directly influence engagement signals that can influence ranking.
Here’s How To Calculate
Metrics
Audience segment size: How many people are in your audience segment? Audience usage size: Number of people who use the ideas in your content. Formula: Adoption rate = (audience usage size/audience segment size) X 100%You can collect this data in a lot of different ways, but shares alone are not a great metric since I don’t believe they reflect actual influence.
Find discussions or actions taken as a result of your ideas or content.
Is your audience discussing your ideas on LinkedIn, Twitter (X), or relevant social? Are newsletters talking about your ideas or the essence of your ideas? Are your process steps being discussed? Do people share videos using your product or ideas?Pro tip: I see some creators concerned about people “stealing” their original idea. I don’t think this is a bad thing. This is a signal of adoption due to the idea of solving a significant problem or opportunity.
Measure 3: Time To Activation
How long does it take your audience to take action on your site?
Time to activation measures how long it takes for your audience to take action by searching a topic or taking action on your site after engaging with your messaging.
These can include brand searches, search keywords you own, document downloads, contacting for a quote, or requesting a demo.
This measure can show how well your content is being adopted or if the messaging aligns with your audience’s journey. Shorter activation times suggest strong alignment with audience needs and higher content efficacy.
How To Measure
Identify an activation point (e.g., events you want the audience to trigger) or goals on the site. Estimate how many people read or engaged with your content. Measure how many people took action around specific events on the site.Pro tip: Some marketers will say you shouldn’t measure your program because attribution modeling doesn’t work or SEO takes time. However, time to activation highlights the importance of evaluating the actions on the site that the campaign should drive. Design campaigns for time to activation of less than 3 months for each event, 6 months for large goals, and 12 months for larger business impacts like creating a new market category.
As you activate your audience, brand search will likely have an impact, as your audience will likely search Google for more information on your topic.
Measure 4: Brand Search Volume
Does the audience search for your brand in search engines?
Brand measures refer to the number of times users search for a specific term you branded or own in search engines.
You can measure this in Google Search Console, searching for your brand name or a term you own.
Pro tip: Brand keywords are reported in Google Analytics under the general search engine (e.g. Google) with non-brand keywords. Look for short-term spikes or sustainable trends in Google Search Console, segmenting it in any way possible (e.g. page, query, date, brand modified term) to find the impact. Design your strategy with the idea of being able to measure brand search impact.
Impact On Your Strategy
Integrating SEO and PR strategies to influence audience behavior and engagement is important for maximizing organic visibility and search rankings.
Google’s recent leaks emphasize the importance of audience engagement, highlighting the need to integrate content creation, SEO, and PR to drive meaningful interactions.
Measures such as asset NPS, idea adoption rate, and time to activation provide valuable insights into audience loyalty, idea adoption, and action times.
These seem to be important for driving engagement and influencing search engine rankings but critical for audience engagement.
These engagement-driven measures will help ensure you don’t have to keep chasing Google’s evolving algorithms and that content genuinely resonates with your audience segment.
Start designing integrated PR and SEO strategies.
More resources:
Experts Vs. Influencers: Digital PR Alternatives To Link Building 7 Steps To Building A Kickass Digital PR Campaign The Dark Side Of Link BuildingFeatured Image: Yurii_Yarema/Shutterstock