Big Data Analytics: The Key to Resolving Complex Business Dilemmas
Big data analytics is the complex process of examining large and diverse datasets to uncover hidden patterns, correlations, market trends, and customer preferences. It is a crucial tool for organizations to make informed business decisions and tackle complex problems....
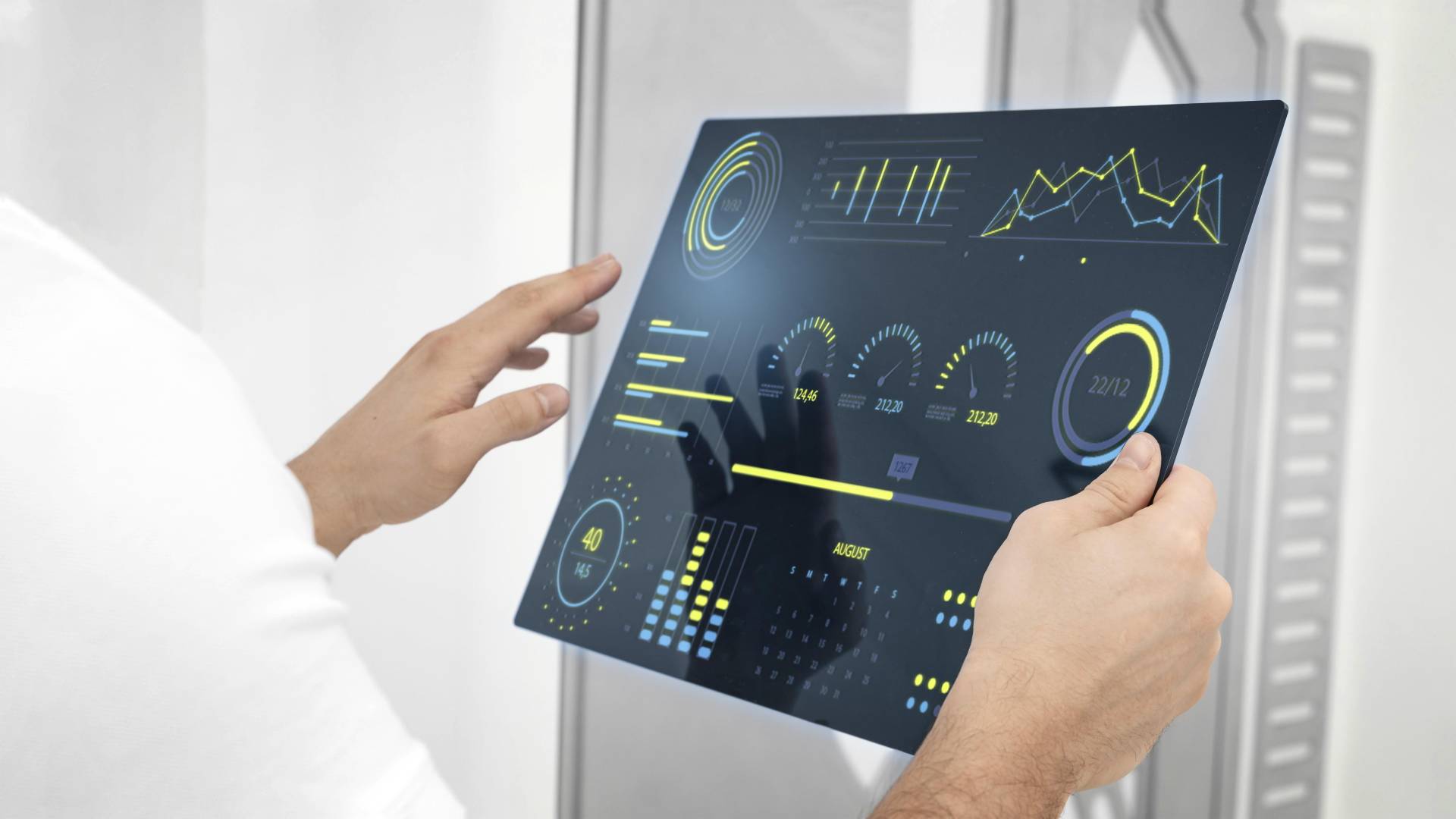
Big data analytics is the complex process of examining large and diverse datasets to uncover hidden patterns, correlations, market trends, and customer preferences. It is a crucial tool for organizations to make informed business decisions and tackle complex problems. In this article, we will explore the significance of big data analytics, its applications, benefits, challenges, and its history and growth.
The Importance of Big Data Analytics
Expertise Matters
Just as you would want a trained physician to diagnose your health problems, you need experts in big data analytics to help solve complex business problems. Subject Matter Experts (SMEs) or Known Opinion Leaders (KOLs) who have proven success in your industry can apply AI and analytics methods to develop a roadmap and lead your organization to success.
Advanced Analytics Techniques
Big data analytics is a form of advanced analytics, which involves complex applications with elements such as predictive models, statistical algorithms, and what-if analyses powered by analytics systems. It differs from traditional business intelligence (BI) queries, which answer basic questions about business operations and performance.
How Big Data Analytics Works
The big data analytics process consists of four main steps:
Data Collection: Data analysts, data scientists, predictive modelers, statisticians, and other analytics professionals collect data from various sources, including semi-structured and unstructured data streams, such as internet clickstream data, web server logs, cloud applications, mobile applications, social media content, text from customer emails and survey responses, mobile phone records, and machine data from IoT sensors. Data Processing: After data is collected and stored in a data warehouse or data lake, data professionals must organize, configure, and partition the data properly for analytical queries. Thorough data preparation and processing lead to higher performance from analytical queries. Data Cleansing: Data professionals scrub the data using scripting tools or data quality software. They look for any errors or inconsistencies, such as duplications or formatting mistakes, and organize and tidy up the data. Data Analysis: The collected, processed, and cleaned data is analyzed with analytics software, which includes tools for data mining, predictive analytics, machine learning, deep learning, text mining, statistical analysis, artificial intelligence (AI), mainstream business intelligence software, and data visualization tools.Key Big Data Analytics Technologies and Tools
Many different types of tools and technologies are used to support big data analytics processes. Some common technologies and tools include:
Hadoop: An open-source framework for storing and processing big data sets, capable of handling large amounts of structured and unstructured data. Predictive Analytics: Hardware and software that process large amounts of complex data and use machine learning and statistical algorithms to make predictions. Stream Analytics: Tools used to filter, aggregate, and analyze big data stored in various formats or platforms. Distributed Storage: Data replicated on a non-relational database, providing protection against node failures and low-latency access. NoSQL Databases: Non-relational data management systems that work well with large sets of distributed data and do not require a fixed schema, making them ideal for raw and unstructured data. Data Lake: A large storage repository that holds native-format raw data until it is needed. Data Warehouse: A repository that stores large amounts of data collected by different sources, using predefined schemas. Knowledge Discovery/Big Data Mining: Tools that enable businesses to mine large amounts of structured and unstructured big data. In-Memory Data Fabric: Distributes large amounts of data across system memory resources, providing low data access and processing latency. Data Virtualization: Enables data access without technical restrictions. Data Integration Software: Streamlines big data across different platforms, including Apache, Hadoop, MongoDB, and Amazon EMR. Data Quality Software: Cleanses and enriches large data sets. Data Preprocessing Software: Prepares data for further analysis, including formatting and cleansing unstructured data. Spark: An open-source cluster computing framework used for batch and stream data processing.Big data analytics applications often include data from both internal systems and external sources, such as weather data or demographic data on consumers compiled by third-party information service providers. Streaming analytics applications are also becoming common in big data environments, as users perform real-time analytics on data fed into Hadoop systems through stream processing engines like Spark, Flink, and Storm.
Big Data Analytics in Various Industries
Big data analytics has been embraced by a diverse range of industries as a key technology driving digital transformation. Users include retailers, financial services firms, insurers, healthcare organizations, manufacturers, energy companies, and other enterprises. Some examples of how big data analytics can be applied in these industries include:
Customer Acquisition and Retention: Consumer data can help companies’ marketing efforts, acting on trends to increase customer satisfaction and create customer loyalty. Targeted Ads: Personalization data from sources such as past purchases, interaction patterns, and product page viewing histories can help generate compelling targeted ad campaigns. Product Development: Big data analytics can provide insights to inform product viability, development decisions, progress measurement, and steer improvements in the direction of what fits a business’s customers. Price Optimization: Retailers may opt for pricing models that use and model data from various sources to maximize revenues. Supply Chain and Channel Analytics: Predictive analytical models can help with preemptive replenishment, B2B supplier networks, inventory management, route optimizations, and the notification of potential delays to deliveries. Risk Management: Big data analytics can identify new risks from data patterns for effective risk management strategies. Improved Decision-Making: Insights extracted from relevant data can help organizations make quicker and better decisions.Benefits of Big Data Analytics
The benefits of using big data analytics services include:
Rapidly analyzing large amounts of data from different sources and formats. Making better-informed decisions for effective strategizing, which can benefit and improve the supply chain, operations, and other areas of strategic decision-making. Cost savings resulting from new business process efficiencies and optimizations. Better understanding of customer needs, behavior, and sentiment, leading to improved marketing insights and valuable information for product development. Improved and better-informed risk management strategies that draw from large sample sizes of data.Challenges of Big Data Analytics
Despite the many benefits that come with using big data analytics, its use also presents challenges:
Accessibility of Data: Storing and processing large amounts of data becomes more complicated as the volume of data increases. Big data should be stored and maintained properly to ensure it can be used by less experienced data scientists and analysts. Data Quality Maintenance: With high volumes of data coming from various sources and in different formats, data quality management for big data requires significant time, effort, and resources. Data Security: The complexity of big data systems presents unique security challenges. Addressing security concerns within such a complicated big data ecosystem can be complex. Choosing the Right Tools: Selecting from the vast array of big data analytics tools and platforms available on the market can be confusing, so organizations must know how to pick the best tool that aligns with users’ needs and infrastructure. Talent Gap: With a potential lack of internal analytics skills and the high cost of hiring experienced data scientists and engineers, some organizations are finding it difficult to fill the gaps.History and Growth of Big Data Analytics
The term “big data” was first used to refer to increasing data volumes in the mid-1990s. In 2001, Doug Laney expanded the definition of big data by describing the increasing volume, variety, and velocity of generated and used data. These three factors became known as the 3Vs of big data. As per recent study most of the routine and daily based task will be automated in 2030.
The launch of the Hadoop distributed processing framework in 2006 was another significant development in the history of big data. Hadoop, an Apache open-source project, laid the foundation for a clustered platform built on top of commodity hardware that could run big data applications.
By 2011, big data analytics began to take a firm hold in organizations and the public eye, along with Hadoop and various related big data technologies. Initially, big data applications were primarily used by large internet and e-commerce companies such as Yahoo, Google, and Facebook, as well as analytics and marketing services providers. More recently, a broader variety of users have embraced big data analytics as a key technology driving digital transformation.
Conclusion
Big data analytics plays a crucial role in addressing complex business problems and helping organizations make informed decisions. Its applications, benefits, and growth have made it an indispensable tool in various industries. By understanding the challenges and choosing the right technologies and tools, organizations can harness the power of big data analytics to drive success and remain competitive in the marketplace.
Maneesh Singh
I am a captivating writer with a unique perspective and an unwavering passion for storytelling. With a talent for weaving words into rich tapestries of imagination, I brings a fresh and engaging voice to the literary world. Drawing inspiration from a myriad of experiences, I crafts compelling narratives that resonate deeply with readers. Their ability to capture the essence of emotions and convey them through the written word is a testament to their innate gift for connecting with others. My writing style is marked by a keen eye for detail, vivid descriptions, and a knack for creating well-rounded characters that readers can't help but root for. They have an uncanny ability to transport readers to new worlds, immersing them in the sights, sounds, and emotions of each story they tell.