The Role of Machine Learning in Data Science: Main Use Cases
Ever wondered how machine learning in data science can come in handy? This informative article will be your in-depth guide […] The post The Role of Machine Learning in Data Science: Main Use Cases appeared first on ReadWrite.
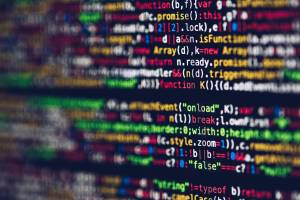
Ever wondered how machine learning in data science can come in handy? This informative article will be your in-depth guide into the complex worlds of data science and machine learning. Below you’ll read about the most common and significant ML applications and the challenges of machine learning in data science.
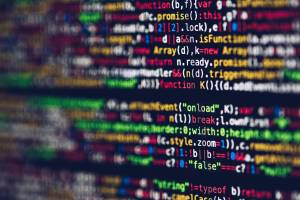
What is Data Science?
Back in the time, businesses collected all types of data in Microsoft Excel, and that was enough. Now, the complexity of data is gradually rising; in fact, according to Forbes, nearly 2.5 quintillion bytes of data are generated every single day. Many businesses and organizations start integrating artificial intelligence into their work pipeline to stay ahead of the competition curve.
Data science is the process of collecting, analyzing, and modeling the data received to identify trends and patterns and extract key insights to benefit the company. For instance, AI-powered recommendation systems can analyze the data representing users’ preferences and make tailored recommendations.
What is Machine Learning?
Machine Learning (ML) is a branch of Artificial Intelligence (AI) that is able to make predictions by learning and evolving upon past experiences and data. It uses algorithms that allow engineers to conduct statistical analyses and draw out patterns in the data. The data can be used in different areas – AI applications are used in a number of important industries, such as insurance, autonomous vehicles, finance and banking, cybersecurity, agriculture, sports, healthcare and telehealth, and many more.
The Role of Machine Learning in Data Science
Machine learning operates on data, and the performance of ML algorithms depends directly on the data quality and quantity of the training data. However, in its turn, modern machine learning technologies enhance and facilitate the data science processes. ML and AI now dominate the field, replacing other Data Science techniques such as ETL and data analytics. ML algorithms use the data by first analyzing chunks of it, then sorting it out into categories or a specific order, and finally making predictions without human intervention. Below we’ll present some of the most commonly used machine learning techniques that are heavily used in data science.
Machine Learning Applications in Data Science
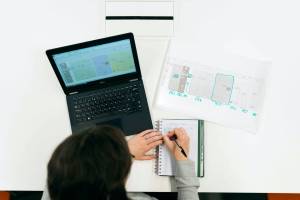
Image Recognition
Image recognition is one of the most prominent and common applications of machine learning in data science. It allows machines to identify objects, humans, and other characteristics in images. Due to a computer vision technique called object detection computers can analyze vast amounts of data, and categorize it based on specific properties. This allows computers to understand the context or further analyze the image and draw conclusions from it. In turn, it allows automation of the business processes, as based on the recognized item, a respective action can be performed.
Speech Recognition
Speech Recognition includes the process of translating verbal discourse into written text. With a simple click, one might exchange words, syllables, and other characters in the text. Various speech recognition algorithms come together to interpret and process human speech, ensuring a low error rate. This opens up a whole new level of data analysis and benefits businesses with a demand for communication investigation, such as call centers, customer support departments, and so on.
Recommendation Algorithms
Online recommendations become increasingly popular with each new online application. Recommendation algorithms allow engines to analyze the users’ experience and, based on the data, make relevant recommendations for the users. Machine learning models observe customer behavior, past purchases, and history to collect relevant data. Recommendation models are especially useful for the product, marketing, customer support, and success teams. On the user side, eCommerce companies are a great example of online recommendation engines with product suggestions for users.
Fraud Detection
Fraud detection has become much easier with the help of machine learning. The models are trained on data to automatically block or allow certain user actions, such as suspicious transactions in the banks, logins into various accounts, and even identifying thefts. Machine learning offers faster detection, reduced manual work, better predictions, and effective solutions.
Main Challenges Machine Learning Poses in Data Science
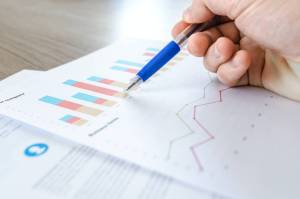
Machine Learning has now been in Data Science for many years and has revolutionized the field entirely. Although ML can be highly beneficial in most cases, there are still some challenges that need to be addressed.
Data Collection
The foundation of every ML model is collecting necessary and reliable data, which, unfortunately, is quite difficult and expensive today. The thing is that almost every company and data scientist has tons of data about almost everything, fluffy stuff included. The abundance of this useless data creates confusion and extends the period to find specific information. Data scientists have to spend hours analyzing and processing the available data and extracting the important ones from the whole mess.
Privacy Limitations
Even if the required data is there, accessing it can still be a bottleneck. Because of the recently increased cyberattacks, more and more organizations are aware of the risks and are taking preventive measures to protect their data. This, of course, is an important factor if we consider privacy concerns, but this also becomes a huge barrier for all the data scientists and experts who try to reach out to the exact dataset that they need.
Inconsistencies in Data
The bad news is that the trained model is not 100% reliable as it has differences between the trained and produced data. The outcome of the trained model may not be as expected and can easily come down based on various factors such as location, mobile device, and even seasonal changes. This is when you should be more attentive and spend enough time regularly updating and improving the model to avoid this challenge as much as possible.
Not Proper Functioning
Machine learning algorithms can function separately without human intervention. This is true, but only partly. However advanced the algorithm becomes, we still need programmers and data scientists to fuel the algorithms to continue creating favorable results. At this point, relying completely on machine learning may not completely solve the problem.
Final Thoughts
In a nutshell, machine learning allows today’s data scientists to gather and analyze vast amounts of data for actionable insights. In addition, it allows businesses to make precise predictions and recommendations. This becomes possible by analyzing historical data and utilizing it in various fields. Machine learning is especially useful for data science when large amounts of data need to be analyzed and categorized. Some common use cases of machine learning in data science include image and speech recognition, relevant online recommendations, and even fraud detection. Though machine learning engineers still may struggle with finding relevant data, accessing private data, or failing to build a model as expected, the technology is revolutionary. With no doubt, machine learning is the best solution for analyzing high volumes of data and offering solutions to increase productivity.