The Power of Artificial Intelligence in Cancer Diagnostics
Artificial intelligence is omnipresent. In the future, queries will be responded to by personal digital assistants, trade stocks will be monitored by robo-advisors, and driverless cars will drive people to their destinations.
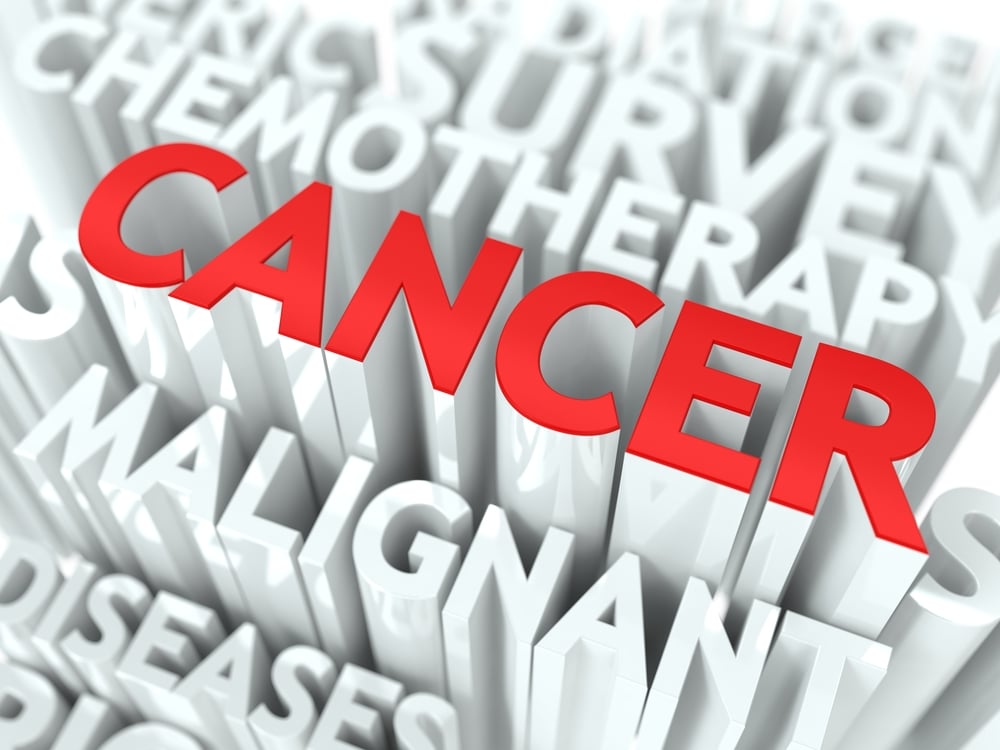
AI is outstanding at acknowledging patterns, sorting through an extensive volume of data, pulling out relationships between intricate attributes, and identifying facets in data that cannot be comprehended by the human brain. Its success and application can already be witnessed in radiology, where clinicians utilize computers to process images speedily, permitting radiologists to concentrate their time on areas where technical understanding is crucial. The vast potential of AI is expected to translate into rapid market growth. The global artificial intelligence in cancer diagnostics market was valued at USD 367.53 million in 2021 and is projected to reach USD 4,841.84 million by 2030, growing at a CAGR of 34.3% during the forecast period, according to Polaris Market Research. Electronic healthcare records: Countless healthcare data procedures are appropriate for analysis with AI. A short time ago, a global electronic healthcare records framework took place, sanctioning extensive amounts of clinical data to be preserved and acquired skillfully. Several digital alliances are working to enhance premature diagnosis research using EHRs. Supplementary digital databases record reaction proceedings and pathway data. For instance, the Digital Cancer Waiting Times Database aspires to enhance cancer benchmark pathways through user-transmitted performance metrics. Radiology: The move from radiographic film to digital scans in Patient Archives and Communication systems (PACS) has created key advantages for imaging research. Radiomics pertains to quantitative methods for analyzing radiology images. This includes CT, nuclear medicine, MRI, and ultrasound scans. Radiomics is divided into conventional ML and DL approaches. For traditional ML, structural attributes are seized from highlighted Regions of Interest (ROI) and assessed for similarities in size and shape, heterogeneity, and intensity readouts. These attributes are utilized to instruct models for categorization. In the premature cancer diagnosis habitat, this involves categorizing undefined nodules or cysts as benign or malignant. Several studies have engaged a radionics approach to segregate nodules precisely in this manner and initiated exact radionics-dependent cancer prospective functions covering several tumor groups such as lung, colorectal, and neck and head cancers. Predictive models have become an essential component in cancer therapy. An individual's probability of developing specific cancers can be determined by predictive models by recognizing risk factors. Medical personnel can then motivate patients to focus on preventive care strategies. Therefore, deep learning can differentiate between the mammograms of women who will subsequently develop breast cancer and those who won’t. Besides mammograms helping identify cancer, this technology can forecast the risk of breast cancer by calculating breast density. While denser breasts call for escalated cancer risk, alternative aspects concealed in mammograms could add to the risk. Machine learning encompasses an extensive range of tasks and methods. Supervised learning exercises have a familiar obtainable result to forecast, such as the appearance of the tumor, length of continuity, or treatment reaction. Unsupervised learning recognizes patterns and subgroups within data without a comprehensible consequence to forecast. It is frequently utilized for more probing analysis. Under supervised learning comes linear models. They plot the unconventional variables to the result of interest through a linear equation. Linear regression presumes that the result linearly conforms to the feature values and that there is an additive connection between features. Linear methods have been a persistent popular option for modeling due to their accountable and straightforward methodology. Such models form the foundation of several overall scores and predictive models utilized throughout healthcare. Nonetheless, results are frequently intrinsically nonlinear in their features. For instance, the influence of tumor size on cancer recurrence probability may vary for contrasting age groups. A linear model does not capture such exchanges between variables. Interaction variables can be structured to mirror nonlinearity. For instance, an individual can generate an acquired feature that merges age and tumor size to model a shared effect. The expeditious growth of computational capability, the growing available of machine-readable EHRs, multi-omics, and medical imaging data, and progressions in DL, especially convolutional neural networks, have transformed the advancement and usage of AI algorithms and CDSS in cancer-associated imaging analysis, genomics, and clinical practice across the cancer continuity. Progressing research to reinforce the application of AI to cancer genomics is expected to aid early detection of multicancer and the establishment of tumor sites. This can transform cancer screening, especially for the less frequent and sparse cancers, and it may change monitoring game plans for cancer survivors. Pursued advances in imaging-dependent ML can cause the advancement of models that gauge risks for several kinds of cancer, devise the diagnostic precision of cancer, or forecast death outcomes. This can permit personalized screening, therapeutic procedures, and survivor monitoring. Additionally, it can reinforce virtual biopsy to classify the genomic and pathologic characteristics linked with cancer diagnostics. For more detailed insights into this fast-growing market, read the comprehensive 112-page report by Polaris Market Research titled Artificial Intelligence in Cancer Diagnostics Market Share, Size, Trends, Industry Analysis, 2022-2030. Polaris Market Research is a market research and consulting organization providing deep-dive market insight to customers around the globe and across industry verticals. Hundreds of reports by this firm are available for purchase on MarketResearch.com.Artificial intelligence is omnipresent. In the future, queries will be responded to by personal digital assistants, trade stocks will be monitored by robo-advisors, and driverless cars will drive people to their destinations. AI has infiltrated the lives of humans, and its usage is also flourishing in biomedical research, where the possible execution for AI is tremendous.
Data Types Used for AI in Cancer Diagnostics
Decision-Making Is Enhanced by Predictive Models
Increasing Use of Machine Learning to Detect Cancer
Recent Developments in AI for Cancer Diagnostics
In August 2022, Whiterabbit.ai endorsed an agreement with Arterys to augment AI generated technology to discover cancer at premature stages.
In July 2022, Roche and EarlySign endorsed an alliance to promote and manage their LungFlag technology. The key aim of their alliance is to detect a premature diagnosis of lung cancer broadly obtainable to the populace.
What’s Next
Where to Learn More
About Polaris Market Research